16
APR
Webinar: Optimizing Cetuximab and winning the 2024 Adaptyv Protein Challenge
16
APR
Webinar: Optimizing Cetuximab and winning the 2024 Adaptyv…
16
APR
Webinar: Optimizing Ce…
8x improvement in EGFR binding affinity: winning the Adaptyv Bio protein design competition
8x improvement in EGFR binding affinity: winning the Adaptyv Bio protein design competition
8x improvement in EGFR binding affinity: winning the Adaptyv Bio protein design competition
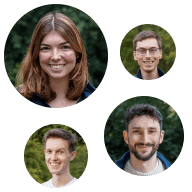
Constance, Jonathan, Gino, Patrick
Constance, Jonathan, Gino, Patrick
December 10, 2024
December 10, 2024



130 teams competed in Adaptyv Bio’s protein design competition to create novel EGFR-binding proteins. Cradle's platform achieved first place with a binding affinity of 1.21nM, which is 8.2 times stronger than Merck's commercial antibody Cetuximab*.
We are proud to announce that Cradle’s submission took the top place in Adaptyv Bio's second protein design competition. The results are in, and we achieved a binding affinity of 1.21nM to EGFR, which is 4x better than the nearest competitor (5.18nM) and 8x better than an scFv-format of Cetuximab (9.94nM) (Erbitux®), a leading cancer therapeutic marketed by Merck.
But best of all: the four of us – Constance, Gino, Jonathan, and Patrick – did the whole thing in about 30 minutes (and over some kombucha). We pressed a few buttons in the Cradle platform, and let it run overnight on our compute cluster. By morning we had the designs, with the whole process requiring zero manual intervention.
The challenge: not your average protein design competition
Adaptyv Bio's competition targeted EGFR (Epidermal Growth Factor Receptor), a high-value therapeutic target.
This transmembrane protein is overexpressed in various cancers including non-small cell lung cancer, colorectal cancer, and head and neck cancer, making it an important target for drugs like Cetuximab (Merck), Panitumumab (Amgen), and Necitumumab (Eli Lilly).
The competition challenged participants to design novel single-chain EGFR binders that had to be at least 10 amino acids different from known therapeutic antibodies. Adaptyv Bio tested the designs in their lab using SPR, validating that the competitors' design strategies translated to real binding.
The competition drew 130 protein designers who submitted 1,131 designs, 400 of which were tested. Many used popular open-source tools like RFdiffusion and BindCraft, while others employed custom solutions. Only two designs out of the 400 tested, including ours, managed to outperform Merck's Cetuximab in terms of binding affinity – highlighting the gap between theoretical possibilities and achieving real-world results.
Optimization over de-novo (structural) approaches
We generated variants through precise framework region modifications and strategic mutation selection.
We began with some quick research to ensure we had all the necessary inputs. Cradle’s strength lies in optimizing existing proteins. With this in mind, we chose scFv-formatted Cetuximab as the starting point and made a strategic decision to block the CDR regions, allowing Cradle’s standard models to focus on optimizing the framework region.
Blocking the CDR regions was critical because these regions are fundamental to the protein's binding function. By protecting the CDRs and directing the platform to optimize the framework, we could preserve the core binding mechanism while finding novel ways to enhance performance. This is in contrast to most traditional antibody engineering, which focuses on CDR optimization. While AI is a powerful tool, applying it effectively requires understanding of the protein engineering space. Knowing which regions to optimize and which to preserve is as important as the computational methods themselves.
After configuring our starting point and selecting which positions to block, we let the platform do what it does best.
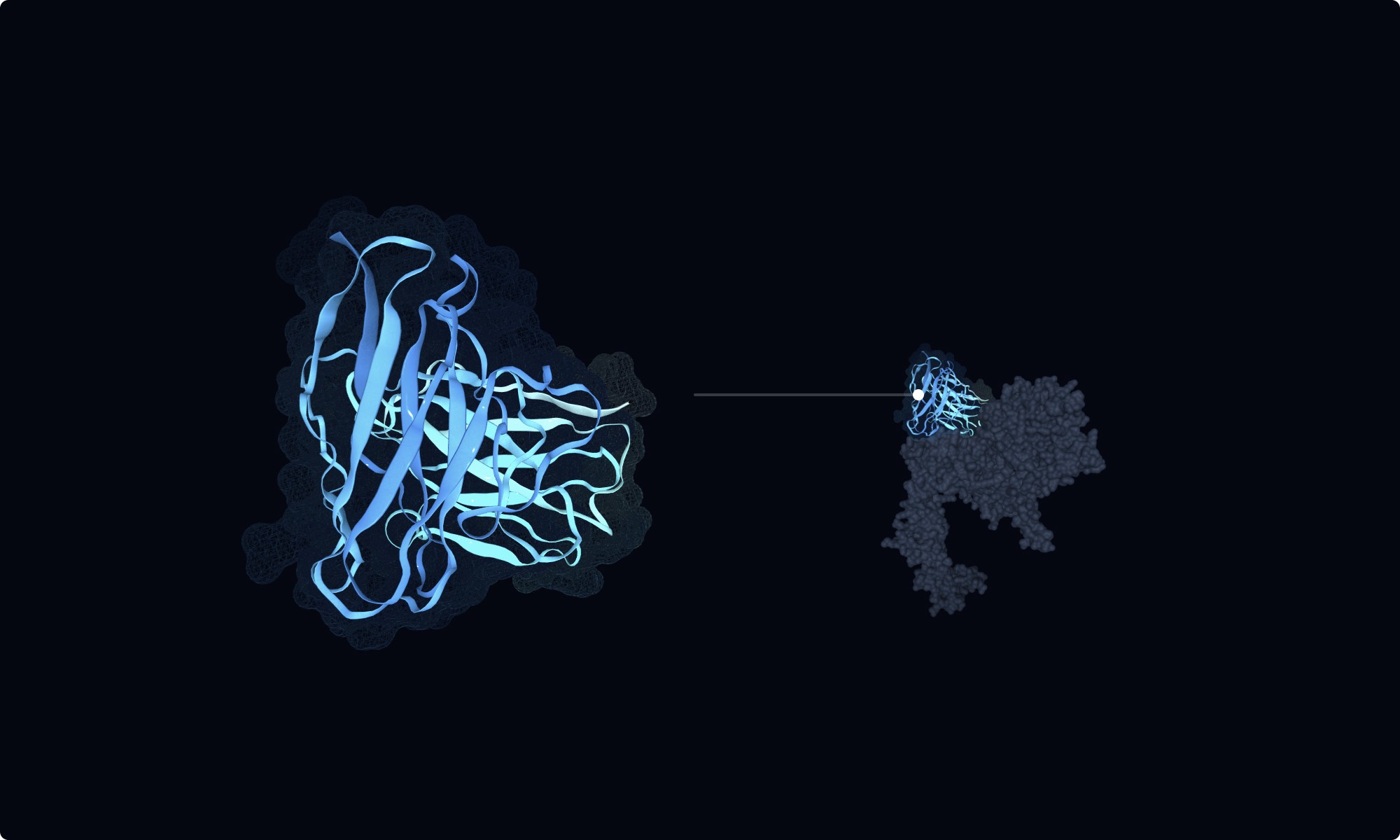
The results: numbers don't lie
Cradle’s design achieved a binding affinity (KD) of 1.21 nM – an 8-fold improvement over Cetuximab scFv (9.94 nM) and 4 times better than the nearest competitor (5.18 nM).
Interestingly, our submission initially ranked between 300-600 on the competition leaderboard due to scoring mechanics that didn't normalize for sequence length. While we identified this issue and reported it on their GitHub (issue), the competition organizers chose to maintain the original scoring to avoid disrupting ongoing submissions.
This highlights a crucial point: in-silico scores don't always tell the whole story. We stayed true to our methodology which is grounded in real-world performance, not optimizing for in-silico scores.
This does however explain why only one of our ten designs advanced to testing, as the organizers needed to distribute their 400-test capacity across more than 1,000 submissions, they used a combination of in-silico rankings and participant representation in their selection process.
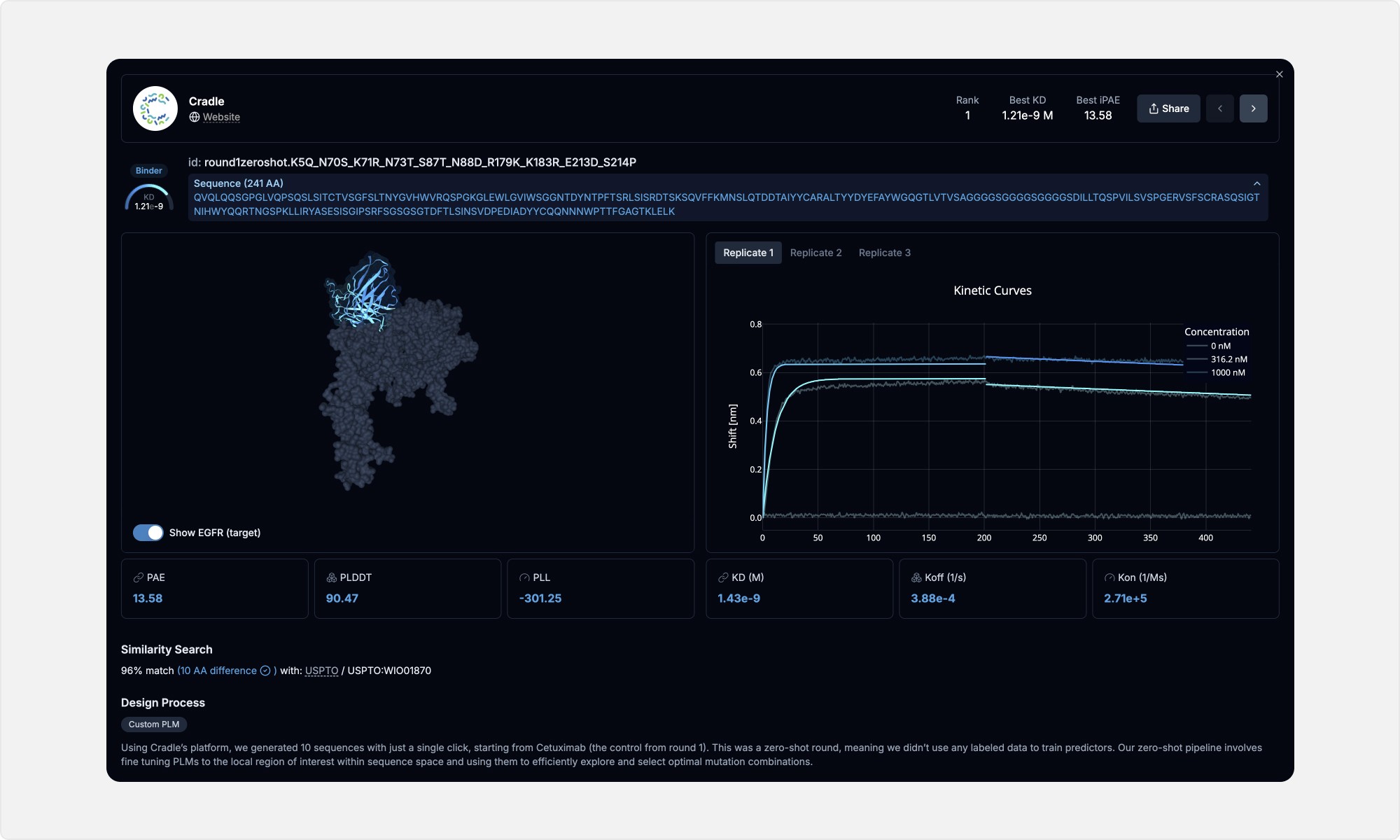
The path to therapeutic development
While we're excited about these results, it's important to understand what this achievement means in the broader context of therapeutic development. Creating a better binder is impressive, but it's one early step in a complex journey. Developing viable drug candidates requires simultaneous optimization across multiple dimensions. The molecule should demonstrate specific target binding and maintain consistent conformational stability across varying thermal and environmental conditions. From a manufacturing perspective, the therapeutic candidate must be amenable to scalable production while retaining its biophysical properties. Crucially, the antibody must exhibit an acceptable immunogenicity profile to ensure patient safety and sustained therapeutic efficacy.
This is where Cradle's full platform capabilities truly shine. This multi-objective optimization challenge is precisely where active learning becomes crucial. By iteratively learning from experimental data, active learning can navigate the complex trade-offs between these properties more effectively than any single-shot approach. Our active learning and optimization pipelines are specifically designed to transform promising initial binders into viable drug candidates through this iterative process.
For those interested in accelerating their protein engineering programs using Cradle's platform, reach out to us here.
Footnotes:
*https://adaptyvbio.substack.com/p/protein-design-competition-results
130 teams competed in Adaptyv Bio’s protein design competition to create novel EGFR-binding proteins. Cradle's platform achieved first place with a binding affinity of 1.21nM, which is 8.2 times stronger than Merck's commercial antibody Cetuximab*.
We are proud to announce that Cradle’s submission took the top place in Adaptyv Bio's second protein design competition. The results are in, and we achieved a binding affinity of 1.21nM to EGFR, which is 4x better than the nearest competitor (5.18nM) and 8x better than an scFv-format of Cetuximab (9.94nM) (Erbitux®), a leading cancer therapeutic marketed by Merck.
But best of all: the four of us – Constance, Gino, Jonathan, and Patrick – did the whole thing in about 30 minutes (and over some kombucha). We pressed a few buttons in the Cradle platform, and let it run overnight on our compute cluster. By morning we had the designs, with the whole process requiring zero manual intervention.
The challenge: not your average protein design competition
Adaptyv Bio's competition targeted EGFR (Epidermal Growth Factor Receptor), a high-value therapeutic target.
This transmembrane protein is overexpressed in various cancers including non-small cell lung cancer, colorectal cancer, and head and neck cancer, making it an important target for drugs like Cetuximab (Merck), Panitumumab (Amgen), and Necitumumab (Eli Lilly).
The competition challenged participants to design novel single-chain EGFR binders that had to be at least 10 amino acids different from known therapeutic antibodies. Adaptyv Bio tested the designs in their lab using SPR, validating that the competitors' design strategies translated to real binding.
The competition drew 130 protein designers who submitted 1,131 designs, 400 of which were tested. Many used popular open-source tools like RFdiffusion and BindCraft, while others employed custom solutions. Only two designs out of the 400 tested, including ours, managed to outperform Merck's Cetuximab in terms of binding affinity – highlighting the gap between theoretical possibilities and achieving real-world results.
Optimization over de-novo (structural) approaches
We generated variants through precise framework region modifications and strategic mutation selection.
We began with some quick research to ensure we had all the necessary inputs. Cradle’s strength lies in optimizing existing proteins. With this in mind, we chose scFv-formatted Cetuximab as the starting point and made a strategic decision to block the CDR regions, allowing Cradle’s standard models to focus on optimizing the framework region.
Blocking the CDR regions was critical because these regions are fundamental to the protein's binding function. By protecting the CDRs and directing the platform to optimize the framework, we could preserve the core binding mechanism while finding novel ways to enhance performance. This is in contrast to most traditional antibody engineering, which focuses on CDR optimization. While AI is a powerful tool, applying it effectively requires understanding of the protein engineering space. Knowing which regions to optimize and which to preserve is as important as the computational methods themselves.
After configuring our starting point and selecting which positions to block, we let the platform do what it does best.
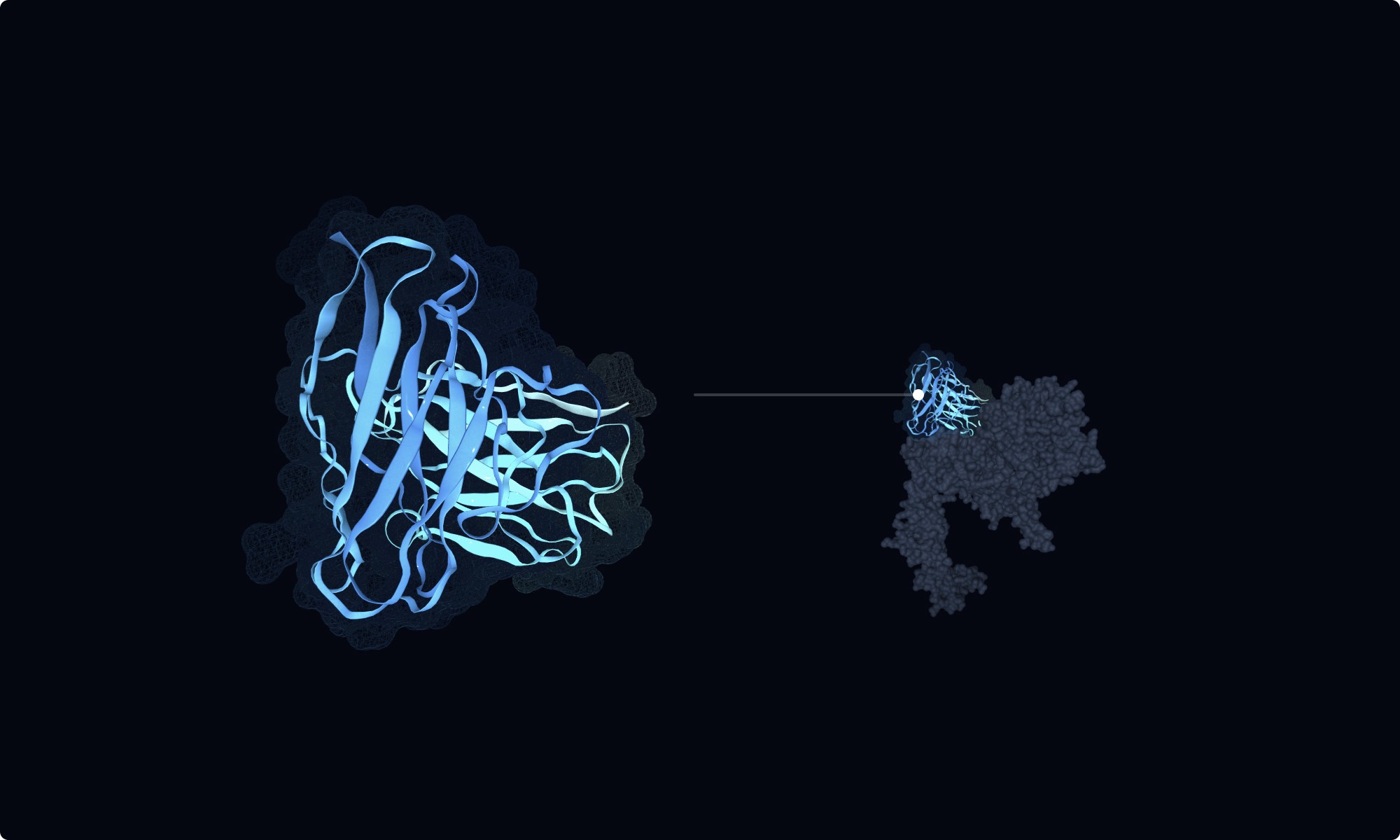
The results: numbers don't lie
Cradle’s design achieved a binding affinity (KD) of 1.21 nM – an 8-fold improvement over Cetuximab scFv (9.94 nM) and 4 times better than the nearest competitor (5.18 nM).
Interestingly, our submission initially ranked between 300-600 on the competition leaderboard due to scoring mechanics that didn't normalize for sequence length. While we identified this issue and reported it on their GitHub (issue), the competition organizers chose to maintain the original scoring to avoid disrupting ongoing submissions.
This highlights a crucial point: in-silico scores don't always tell the whole story. We stayed true to our methodology which is grounded in real-world performance, not optimizing for in-silico scores.
This does however explain why only one of our ten designs advanced to testing, as the organizers needed to distribute their 400-test capacity across more than 1,000 submissions, they used a combination of in-silico rankings and participant representation in their selection process.
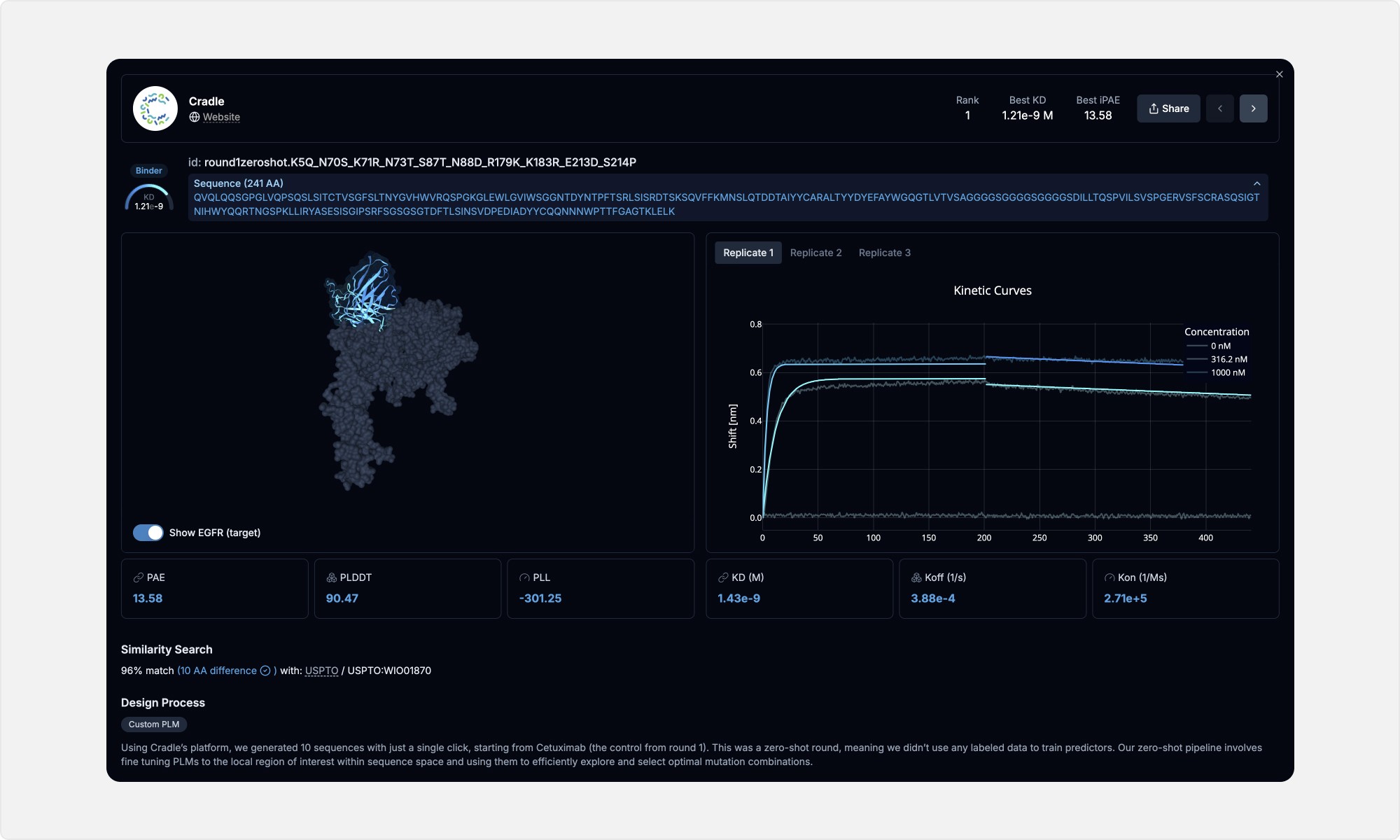
The path to therapeutic development
While we're excited about these results, it's important to understand what this achievement means in the broader context of therapeutic development. Creating a better binder is impressive, but it's one early step in a complex journey. Developing viable drug candidates requires simultaneous optimization across multiple dimensions. The molecule should demonstrate specific target binding and maintain consistent conformational stability across varying thermal and environmental conditions. From a manufacturing perspective, the therapeutic candidate must be amenable to scalable production while retaining its biophysical properties. Crucially, the antibody must exhibit an acceptable immunogenicity profile to ensure patient safety and sustained therapeutic efficacy.
This is where Cradle's full platform capabilities truly shine. This multi-objective optimization challenge is precisely where active learning becomes crucial. By iteratively learning from experimental data, active learning can navigate the complex trade-offs between these properties more effectively than any single-shot approach. Our active learning and optimization pipelines are specifically designed to transform promising initial binders into viable drug candidates through this iterative process.
For those interested in accelerating their protein engineering programs using Cradle's platform, reach out to us here.
Footnotes:
*https://adaptyvbio.substack.com/p/protein-design-competition-results


Optimizing Cetuximab
Optimizing Cetuximab
Optimizing Cetuximab
Mar 31, 2025
Mar 31, 2025


Next-level high throughput workflows: Gotta tag 'em all
Next-level high throughput workflows: Gotta tag 'em all
Next-level high throughput workflows: Gotta tag 'em all
Mar 6, 2025
Mar 6, 2025


Cradle Announces Launch of Advanced Biotechnology Report with Industry Coalition
Cradle Announces Launch of Advanced Biotechnology Report with Industry Coalition
Cradle Announces Launch of Advanced Biotechnology Report with Industry Coalition
Mar 11, 2025
Mar 11, 2025


SLAS 2025: A Peek at The Future of Lab Automation
SLAS 2025: A Peek at The Future of Lab Automation
SLAS 2025: A Peek at The Future of Lab Automation
Feb 26, 2025
Feb 26, 2025


8x improvement in EGFR binding affinity: winning the Adaptyv Bio protein design competition
8x improvement in EGFR binding affinity: winning the Adaptyv Bio protein design competition
8x improvement in EGFR binding affinity: winning the Adaptyv Bio protein design competition
Dec 10, 2024
Dec 10, 2024

Stay in the loop
Stay in the loop
Stay in the loop
Get new posts and other Cradle updates directly to your inbox. No spam :)