'Align to Innovate' benchmark: state-of-the-art enzyme engineering with fully-automated GenAI
'Align to Innovate' benchmark: state-of-the-art enzyme engineering with fully-automated GenAI
'Align to Innovate' benchmark: state-of-the-art enzyme engineering with fully-automated GenAI
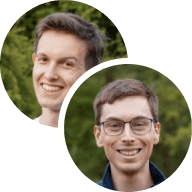
Patrick Kidger & Gino Brunner
Patrick Kidger & Gino Brunner
October 3, 2024
October 3, 2024
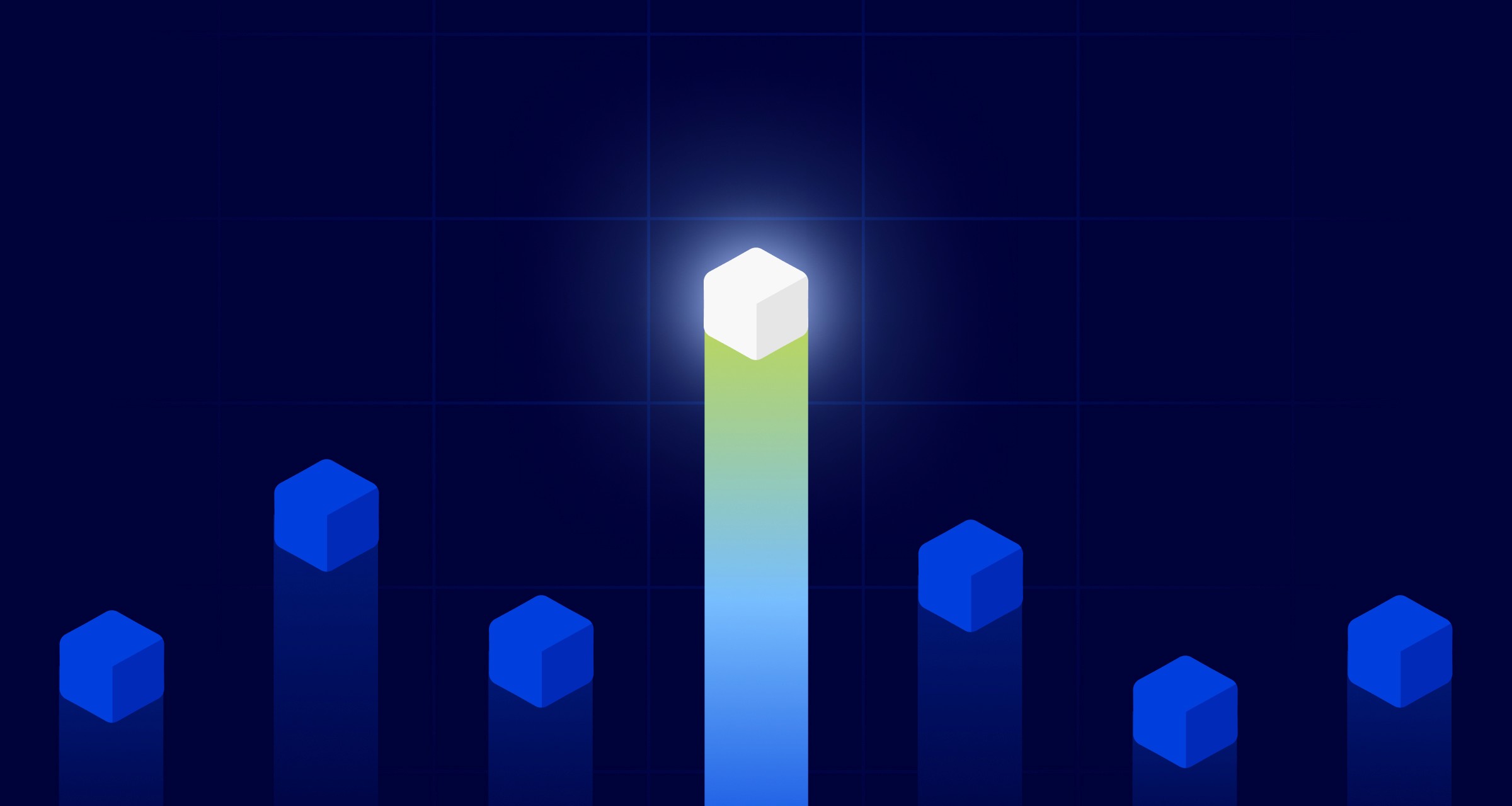
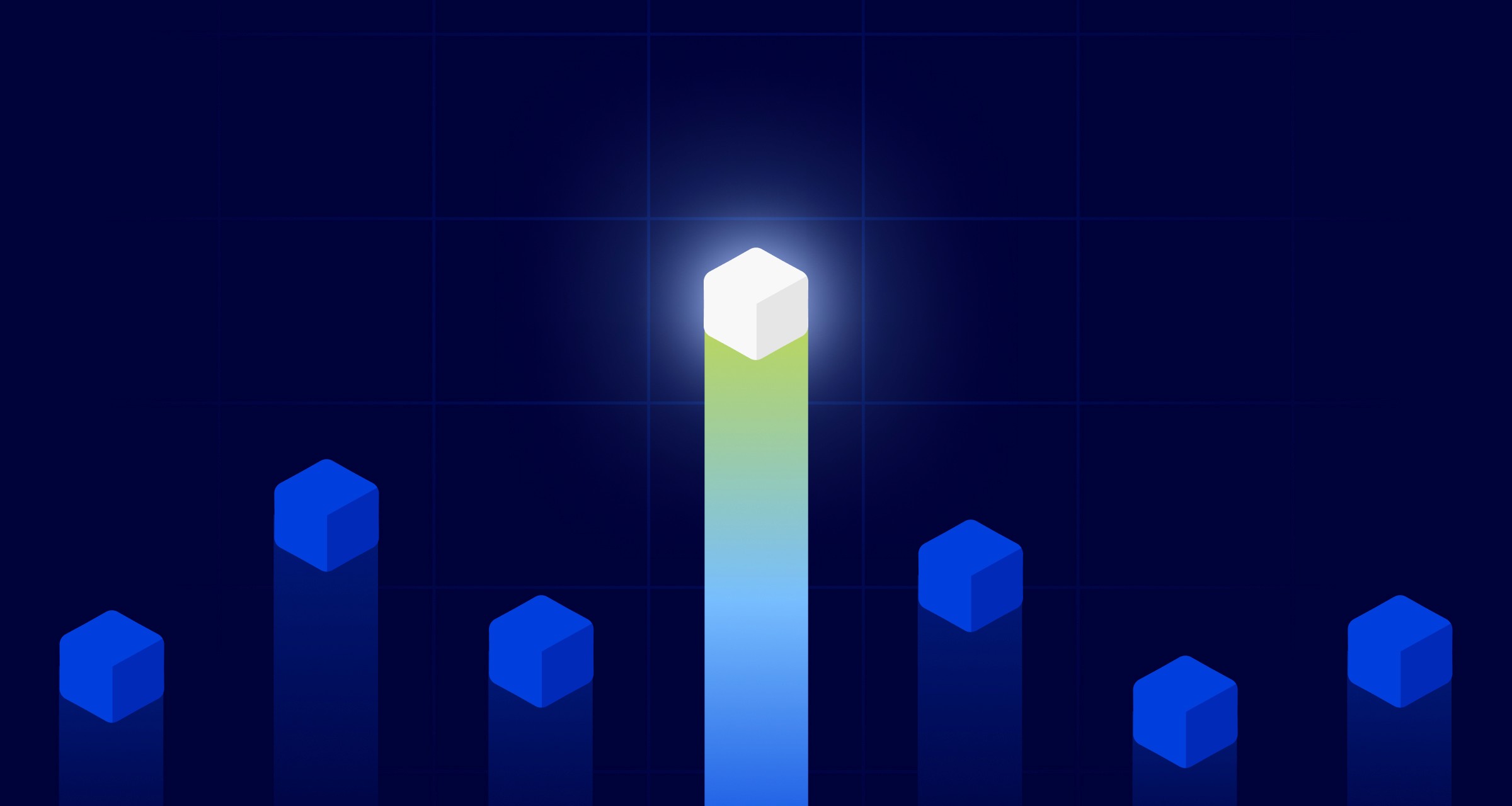
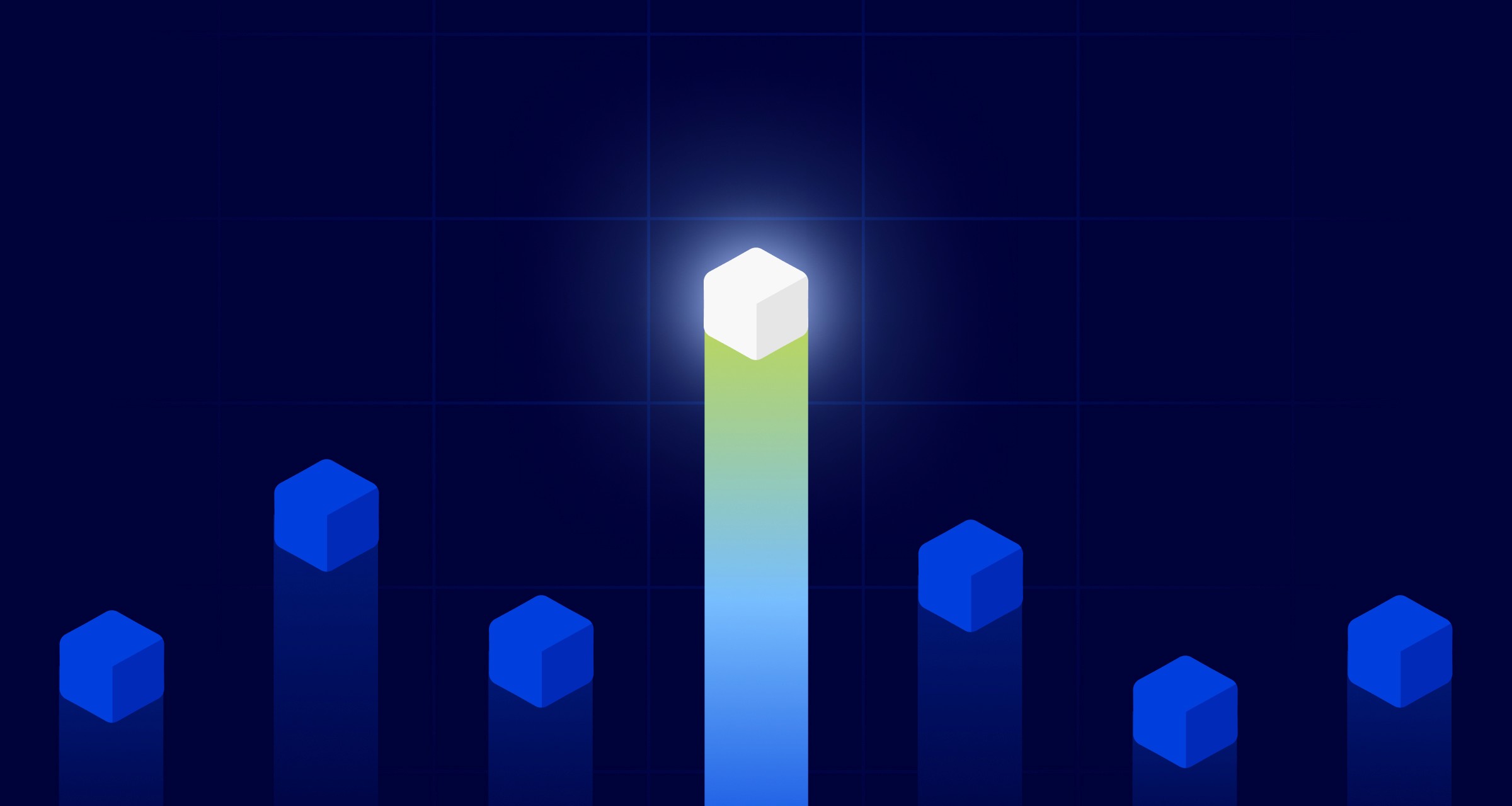
TL;DR: Here at Cradle, we've built an AI platform for discovering and lead-optimizing proteins. We found that we were able to achieve state of the art on the enzyme optimization challenges of the ‘Align to Innovate’ benchmark with our automatically generated models.
Align to Innovate
The ‘Align to Innovate’ tournament was designed to benchmark the latest computational methods in enzyme engineering, in scenarios that closely mimic real-world challenges, and featured over 30 teams from industry and academia [1]. While Cradle did not participate in the tournament at the time, we were recently asked by a potential customer to run the benchmark to see how we would stack up – and we’re excited about the results! Across the four supervised enzyme property prediction challenges, our automatically generated models twice beat first place, and twice tied first place.
What makes these results particularly noteworthy is that we achieved them using only our platform's auto-mode, showcasing the powerful out-of-the-box performance of our fully automated process. With zero human intervention, we matched or outperformed best-in-class methods that typically require extensive custom tuning and feature engineering. While our platform offers the flexibility to manually input numerous variables, many users find the auto-mode sufficient for most applications. This approach allows protein engineers to strategically oversee the optimization process, intervening only when their expert knowledge suggests a potential advantage.
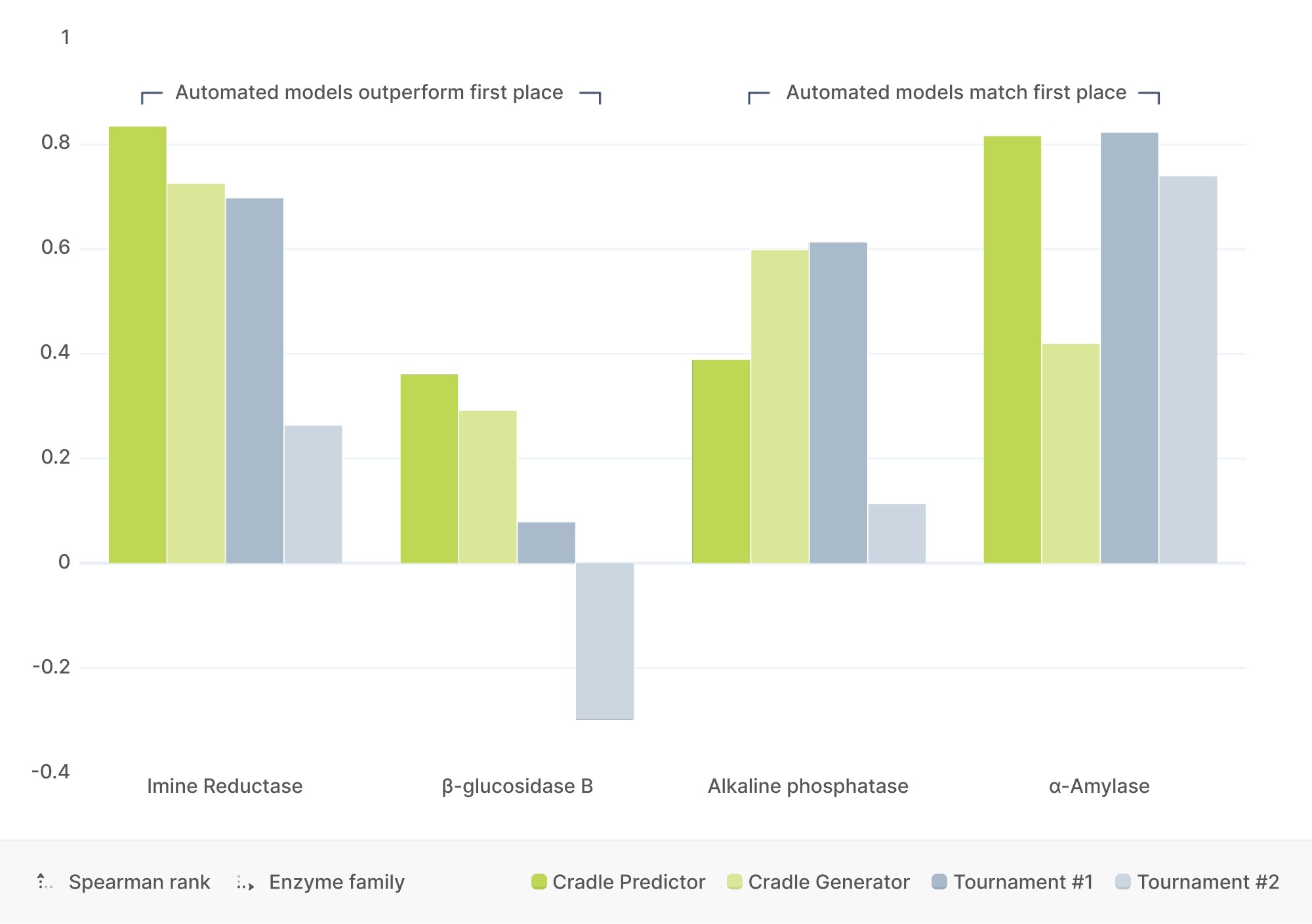
Automated protein engineering
At Cradle, we see early evidence that AI for protein engineering has graduated from being an academic research topic: it has become a reliable technique, suitable for integration into real-world enzyme engineering workflows. As such, we build a fully-automated Gen-AI platform to robustly optimize multiple properties, across multiple protein modalities, without requiring time-consuming manual interventions. This automation enables widespread adoption of the technology across many simultaneous projects, whether that’s at biotech startups or at global pharmaceutical companies.
The results
The 'Align to Innovate' challenge presents data on four different enzyme families: alkaline phosphatase, α-amylase, β-glucosidase B, and imine reductase. For each enzyme, the data includes protein sequences and their corresponding experimental readouts. The task was to correctly predict the ranking of activity, expression, and thermostability for each enzyme family, from worst to best, using a held-out test set [2]. Performance was evaluated using Spearman rank [3], where a higher value indicates that a model is better at correctly ordering protein sequences from best to worst.
Our models demonstrated exceptional performance across all four enzymes in the challenge, twice outperforming and twice matching the tournament winner. On β-glucosidase B, our best model achieved a Spearman rank of 0.36 – this substantially outperforms all competitors, whose scores ranged from 0.08 to -0.3. This was rated as the most challenging enzyme to predict in the challenge.
On imine reductase, β-glucosidase B, and alkaline phosphatase, we observe something else interesting: that specifically our ‘generator’-type models exhibit strong performance. Let’s unpack that: time for a little inside baseball.
As part of Cradle’s usual protein engineering process, we actually train several different kinds of models that work together. One group we call ‘predictors’, and they evaluate sequence quality for different properties / assays and help us down-select to ensure diversity. (You can see them in the graph as well.) Another group we call ‘generators’, and these suggest new protein variants. We find that the in silico Spearman rank of a generator is a strong proxy for its real-world performance.
As such, that result is quite exciting. A generator that ranks proteins accurately in our computational tests is likely to produce valuable candidates for experimental testing, enhancing the efficiency of our entire protein optimization process.
Conclusion: Cradle’s AI platform for protein design
Cradle offers a software platform for AI-guided lead optimization of proteins for therapeutic, diagnostic, chemical, agricultural and food applications. We’re already used by clients across pharma, biotech, agritech, foodtech, and academia – and they’ve reported speedups of 1.5× to 12× (as measured by number of experimental wet lab rounds), along with cost savings of millions of dollars per project. Our platform is fully automated, targets multiple modalities (antibodies, enzymes, vaccines, cytokines, recombinant proteins, etc.), performs simultaneous multi-property discovery and optimization (affinity, expression, …) and the AI models improve performance round-over-round.
This is offered for a predictable subscription fee. We don’t claim royalties or milestones, and full IP rights stay with customers. Cradle leverages its unique position – with clients from across the industry – to develop best-in-class AI techniques, enabling clients to take on more projects, derisk faster, and pursue more ambitious goals. Request an invite.

Appendix: Technical details
Modeling
For this challenge we ran our workflows for fine-tuning generative and predictive models. (Each property has a separate ranking.) Train set sizes ranged from 90 to 10800 sequences, and test set sizes ranged from 30 to 570.
For each enzyme, our automated pipelines begin by running mmseqs [4] to retrieve and align homologous sequences, and fine-tune a foundation model on this evolutionary context. The size of the context is determined automatically by considering e-value, whilst the set of fine-tuning techniques have been determined through ablation on our past studies (both in-house and with our partners). We refer to the result as the ‘base model’: it has been trained on evolutionary information but has not yet seen in-domain data.
Here, the automated pipeline forks: one copy of the base model is now fine-tuned via preference-based optimization on the in-domain training labels; we refer to the result as the ‘generators’. Meanwhile a separate ensemble is fine-tuned using ranking losses to obtain an ensemble of ‘predictors’.
Later steps then typically include generating candidate sequences, scoring them, downselecting them (by letting the generators and predictors interact), round-over-round active learning criteria, structure prediction, molecular dynamics simulation, visualization, reporting, … all orchestrated automatically. But for this in silico challenge, we skip all of that and directly evaluate the models on the test sets.
Spearman rank: a rule of thumb
For reference, in AI for protein engineering, our rule of thumb is that a Spearman rank of at least 0.4 is required for a model to be useful, and at least 0.7 is required to be considered ‘good’. For this evaluation, we used the generator and the base model as rankers by sorting their pseudo-log-likelihood scores [5]. (Meanwhile the predictors directly output ranks.)
FAQ: in-domain vs out-of-domain?
This post has focused on fine-tuning foundation models to exhibit state of the art performance within a local region of the protein landscape. Those following the AI-for-proteins literature may be a little surprised by our focus on this, as so much of the research is focusing on out-of-domain generalization.
This is a great research question, and we love watching progress on this. However what Cradle uniquely demonstrates is that with a wet-lab in the loop, we can transform ‘out of domain’ to ‘in domain’ with very few data points (typically one 96-well plate is enough to get good performance), transforming these protein optimization problems from ‘current tricky research question’ to ‘reliable engineering’.
Other challenges
The eagle-eyed amongst you may have spotted that 'Align to innovate' also included a few other challenges, for example an in vitro round. We did not do a comparison for the other challenges, as it would have been hard to do a fair comparison. (For example, whilst we do have an in-house wet lab, it is notoriously difficult to set up an in-vitro experiment the same way in two different labs.)
TL;DR: Here at Cradle, we've built an AI platform for discovering and lead-optimizing proteins. We found that we were able to achieve state of the art on the enzyme optimization challenges of the ‘Align to Innovate’ benchmark with our automatically generated models.
Align to Innovate
The ‘Align to Innovate’ tournament was designed to benchmark the latest computational methods in enzyme engineering, in scenarios that closely mimic real-world challenges, and featured over 30 teams from industry and academia [1]. While Cradle did not participate in the tournament at the time, we were recently asked by a potential customer to run the benchmark to see how we would stack up – and we’re excited about the results! Across the four supervised enzyme property prediction challenges, our automatically generated models twice beat first place, and twice tied first place.
What makes these results particularly noteworthy is that we achieved them using only our platform's auto-mode, showcasing the powerful out-of-the-box performance of our fully automated process. With zero human intervention, we matched or outperformed best-in-class methods that typically require extensive custom tuning and feature engineering. While our platform offers the flexibility to manually input numerous variables, many users find the auto-mode sufficient for most applications. This approach allows protein engineers to strategically oversee the optimization process, intervening only when their expert knowledge suggests a potential advantage.
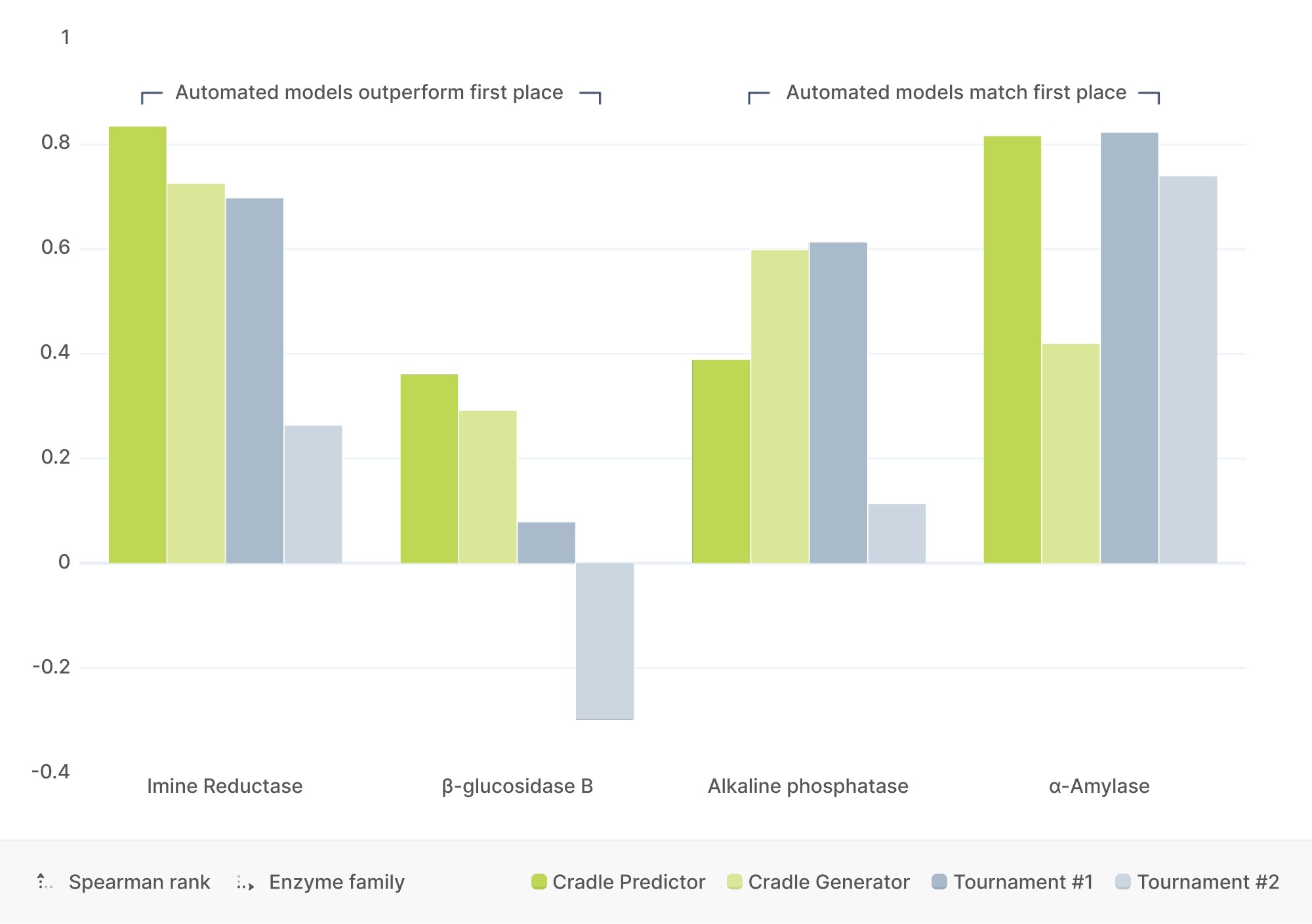
Automated protein engineering
At Cradle, we see early evidence that AI for protein engineering has graduated from being an academic research topic: it has become a reliable technique, suitable for integration into real-world enzyme engineering workflows. As such, we build a fully-automated Gen-AI platform to robustly optimize multiple properties, across multiple protein modalities, without requiring time-consuming manual interventions. This automation enables widespread adoption of the technology across many simultaneous projects, whether that’s at biotech startups or at global pharmaceutical companies.
The results
The 'Align to Innovate' challenge presents data on four different enzyme families: alkaline phosphatase, α-amylase, β-glucosidase B, and imine reductase. For each enzyme, the data includes protein sequences and their corresponding experimental readouts. The task was to correctly predict the ranking of activity, expression, and thermostability for each enzyme family, from worst to best, using a held-out test set [2]. Performance was evaluated using Spearman rank [3], where a higher value indicates that a model is better at correctly ordering protein sequences from best to worst.
Our models demonstrated exceptional performance across all four enzymes in the challenge, twice outperforming and twice matching the tournament winner. On β-glucosidase B, our best model achieved a Spearman rank of 0.36 – this substantially outperforms all competitors, whose scores ranged from 0.08 to -0.3. This was rated as the most challenging enzyme to predict in the challenge.
On imine reductase, β-glucosidase B, and alkaline phosphatase, we observe something else interesting: that specifically our ‘generator’-type models exhibit strong performance. Let’s unpack that: time for a little inside baseball.
As part of Cradle’s usual protein engineering process, we actually train several different kinds of models that work together. One group we call ‘predictors’, and they evaluate sequence quality for different properties / assays and help us down-select to ensure diversity. (You can see them in the graph as well.) Another group we call ‘generators’, and these suggest new protein variants. We find that the in silico Spearman rank of a generator is a strong proxy for its real-world performance.
As such, that result is quite exciting. A generator that ranks proteins accurately in our computational tests is likely to produce valuable candidates for experimental testing, enhancing the efficiency of our entire protein optimization process.
Conclusion: Cradle’s AI platform for protein design
Cradle offers a software platform for AI-guided lead optimization of proteins for therapeutic, diagnostic, chemical, agricultural and food applications. We’re already used by clients across pharma, biotech, agritech, foodtech, and academia – and they’ve reported speedups of 1.5× to 12× (as measured by number of experimental wet lab rounds), along with cost savings of millions of dollars per project. Our platform is fully automated, targets multiple modalities (antibodies, enzymes, vaccines, cytokines, recombinant proteins, etc.), performs simultaneous multi-property discovery and optimization (affinity, expression, …) and the AI models improve performance round-over-round.
This is offered for a predictable subscription fee. We don’t claim royalties or milestones, and full IP rights stay with customers. Cradle leverages its unique position – with clients from across the industry – to develop best-in-class AI techniques, enabling clients to take on more projects, derisk faster, and pursue more ambitious goals. Request an invite.

Appendix: Technical details
Modeling
For this challenge we ran our workflows for fine-tuning generative and predictive models. (Each property has a separate ranking.) Train set sizes ranged from 90 to 10800 sequences, and test set sizes ranged from 30 to 570.
For each enzyme, our automated pipelines begin by running mmseqs [4] to retrieve and align homologous sequences, and fine-tune a foundation model on this evolutionary context. The size of the context is determined automatically by considering e-value, whilst the set of fine-tuning techniques have been determined through ablation on our past studies (both in-house and with our partners). We refer to the result as the ‘base model’: it has been trained on evolutionary information but has not yet seen in-domain data.
Here, the automated pipeline forks: one copy of the base model is now fine-tuned via preference-based optimization on the in-domain training labels; we refer to the result as the ‘generators’. Meanwhile a separate ensemble is fine-tuned using ranking losses to obtain an ensemble of ‘predictors’.
Later steps then typically include generating candidate sequences, scoring them, downselecting them (by letting the generators and predictors interact), round-over-round active learning criteria, structure prediction, molecular dynamics simulation, visualization, reporting, … all orchestrated automatically. But for this in silico challenge, we skip all of that and directly evaluate the models on the test sets.
Spearman rank: a rule of thumb
For reference, in AI for protein engineering, our rule of thumb is that a Spearman rank of at least 0.4 is required for a model to be useful, and at least 0.7 is required to be considered ‘good’. For this evaluation, we used the generator and the base model as rankers by sorting their pseudo-log-likelihood scores [5]. (Meanwhile the predictors directly output ranks.)
FAQ: in-domain vs out-of-domain?
This post has focused on fine-tuning foundation models to exhibit state of the art performance within a local region of the protein landscape. Those following the AI-for-proteins literature may be a little surprised by our focus on this, as so much of the research is focusing on out-of-domain generalization.
This is a great research question, and we love watching progress on this. However what Cradle uniquely demonstrates is that with a wet-lab in the loop, we can transform ‘out of domain’ to ‘in domain’ with very few data points (typically one 96-well plate is enough to get good performance), transforming these protein optimization problems from ‘current tricky research question’ to ‘reliable engineering’.
Other challenges
The eagle-eyed amongst you may have spotted that 'Align to innovate' also included a few other challenges, for example an in vitro round. We did not do a comparison for the other challenges, as it would have been hard to do a fair comparison. (For example, whilst we do have an in-house wet lab, it is notoriously difficult to set up an in-vitro experiment the same way in two different labs.)
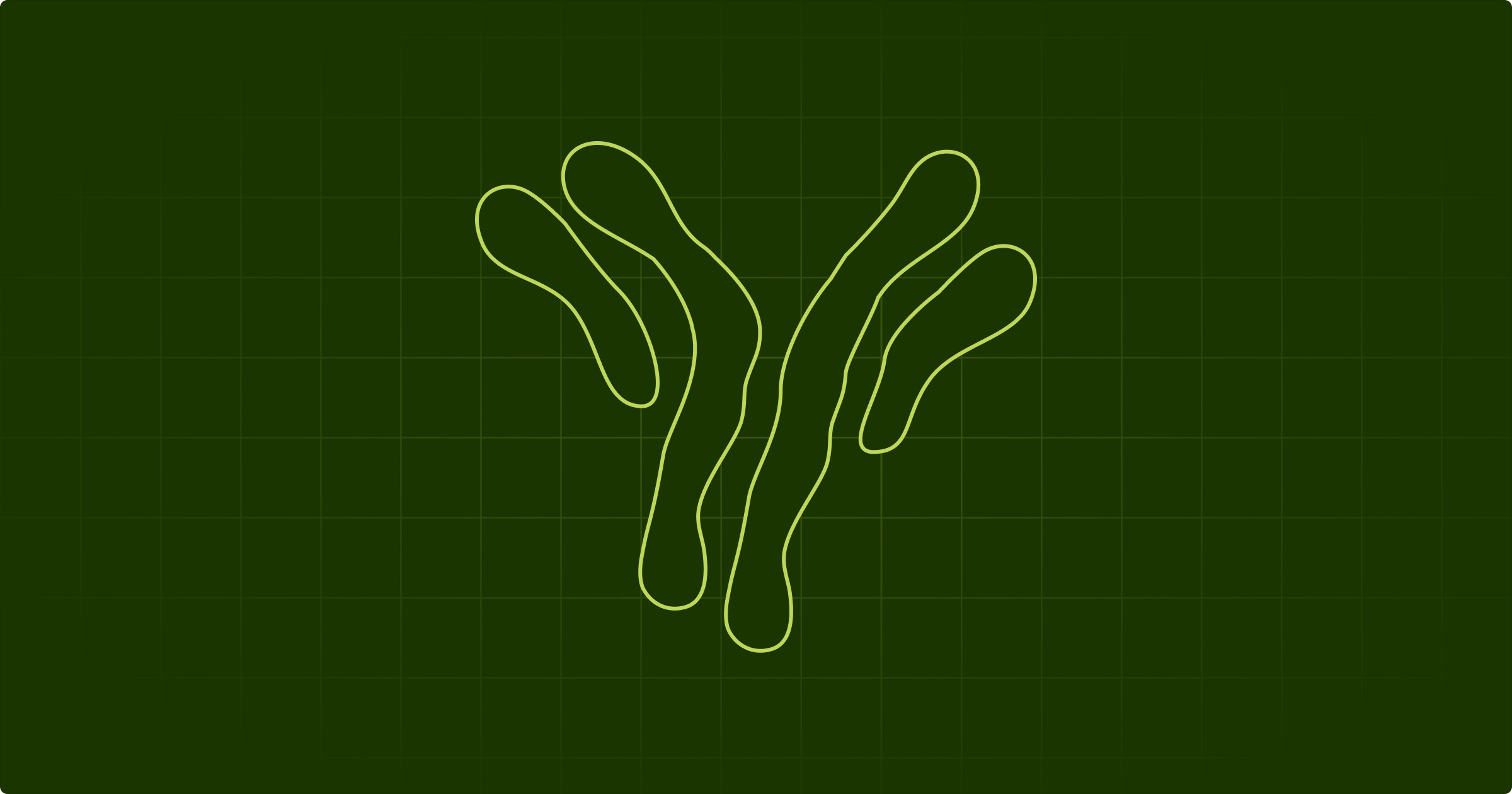
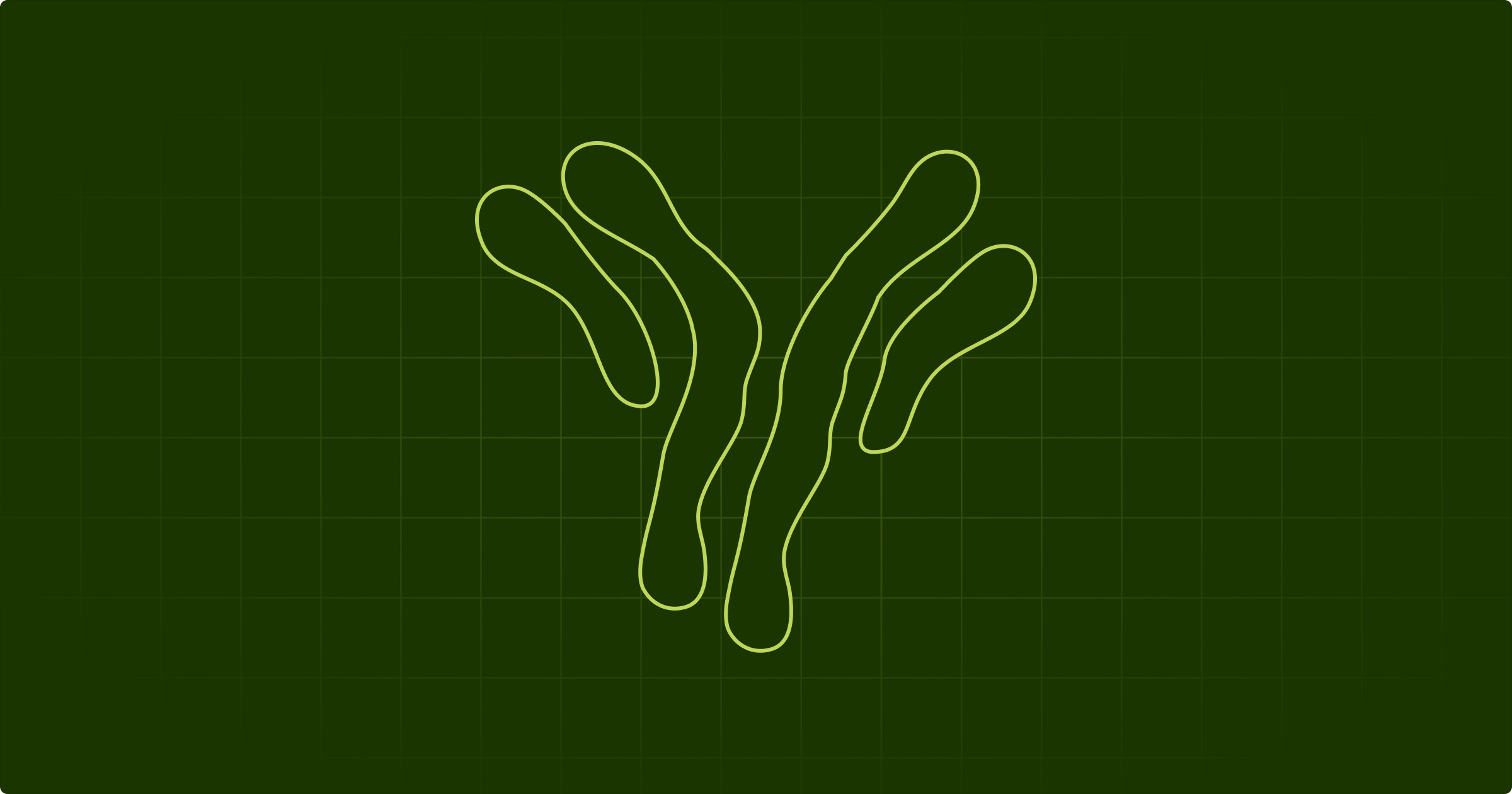
Our Initiative to Accelerate Antibody Discovery
Our Initiative to Accelerate Antibody Discovery
Our Initiative to Accelerate Antibody Discovery
Nov 11, 2024
Nov 11, 2024
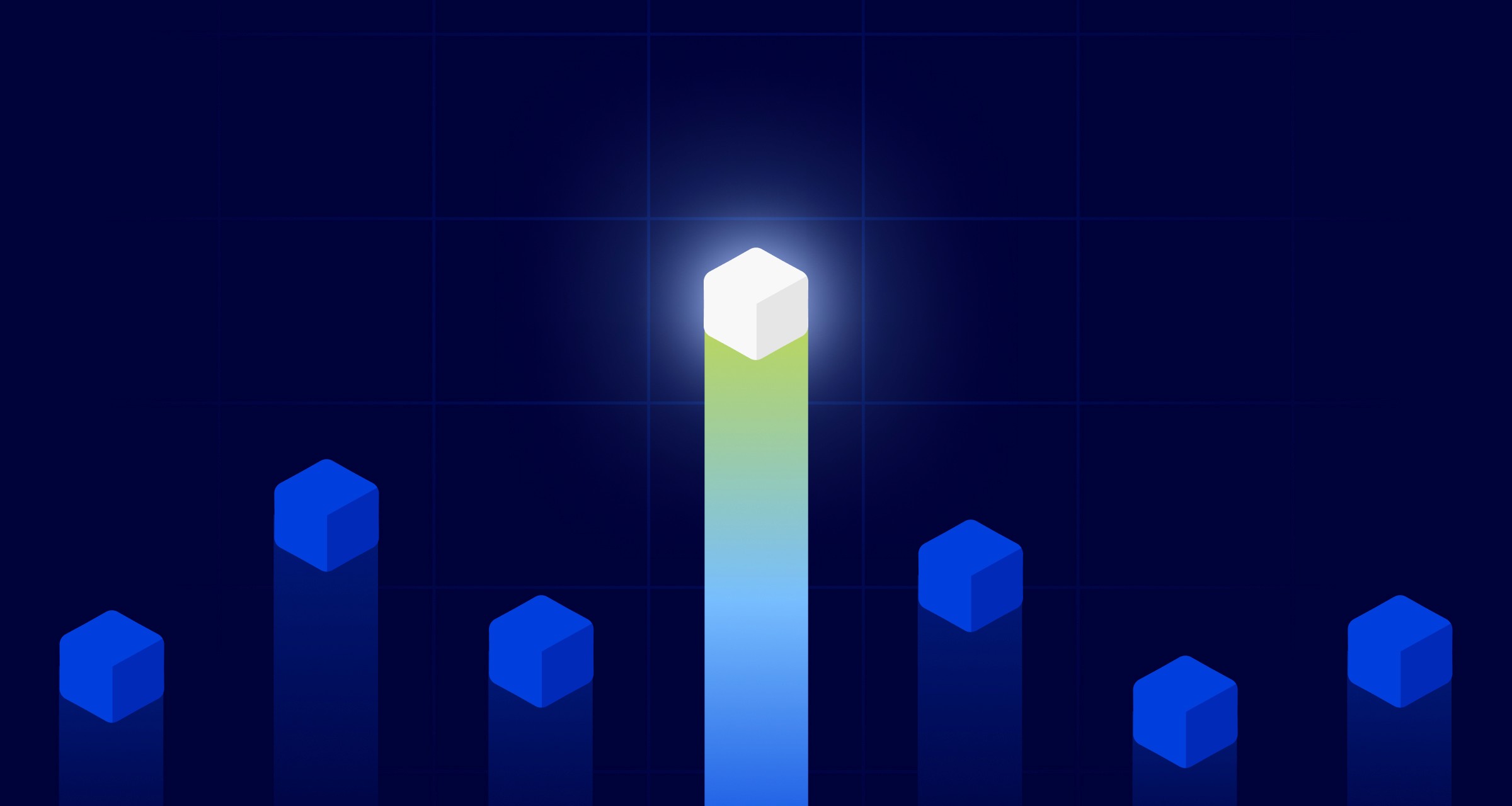
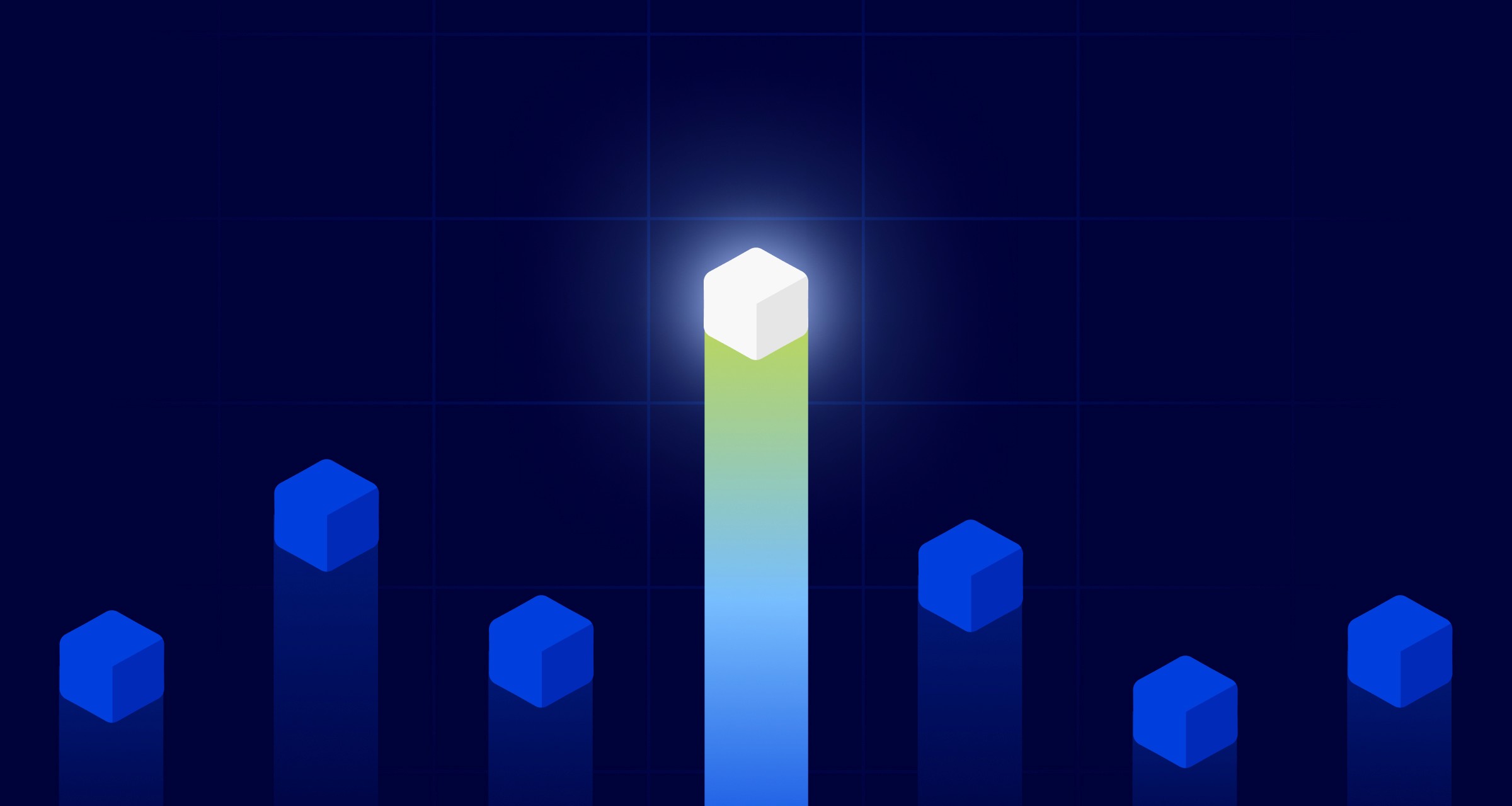
'Align to Innovate' benchmark: state-of-the-art enzyme engineering with fully-automated GenAI
'Align to Innovate' benchmark: state-of-the-art enzyme engineering with fully-automated GenAI
'Align to Innovate' benchmark: state-of-the-art enzyme engineering with fully-automated GenAI
Oct 3, 2024
Oct 3, 2024
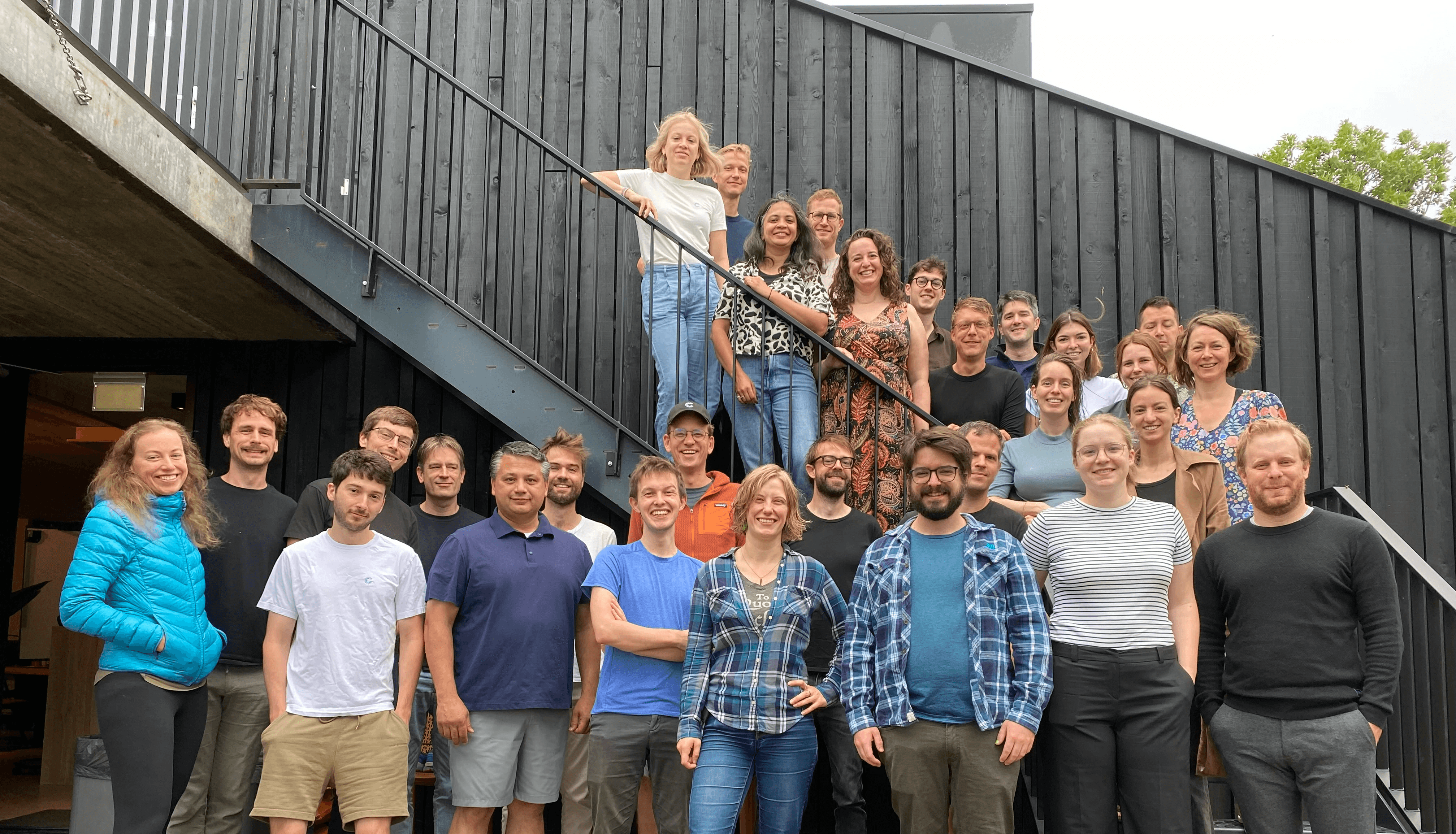
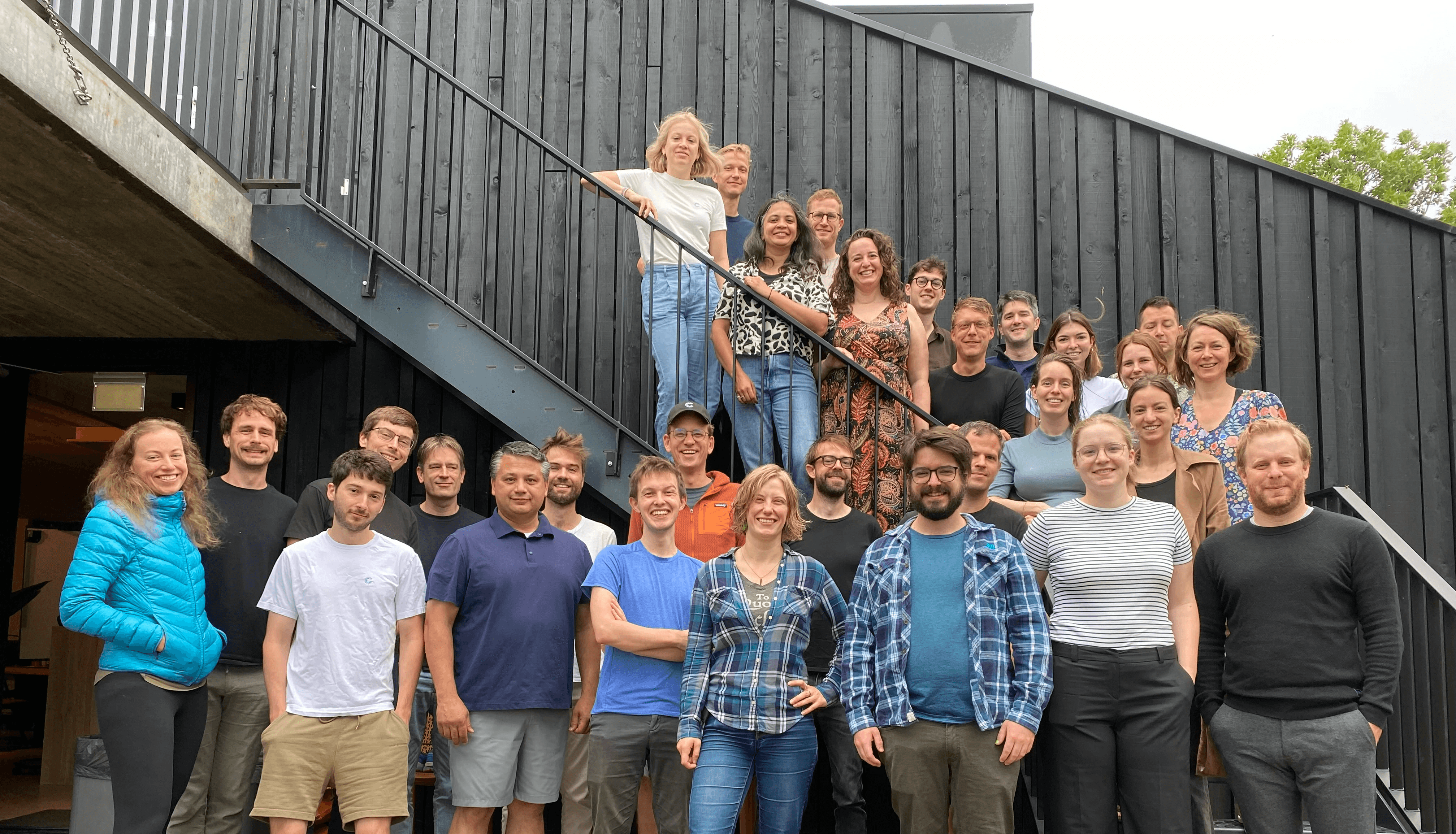
Cultural values at Cradle
Cultural values at Cradle
Cultural values at Cradle
Oct 2, 2024
Oct 2, 2024
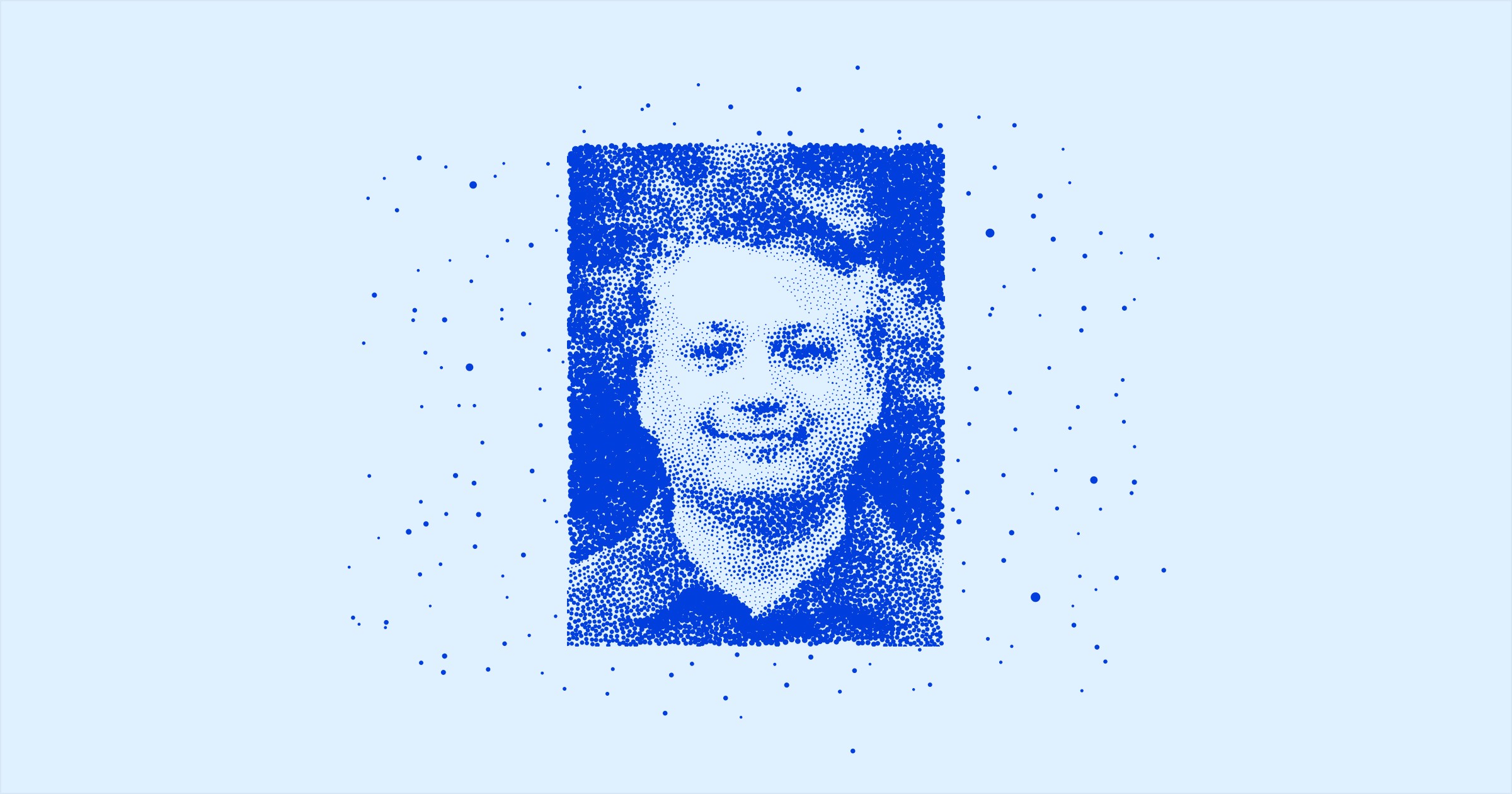
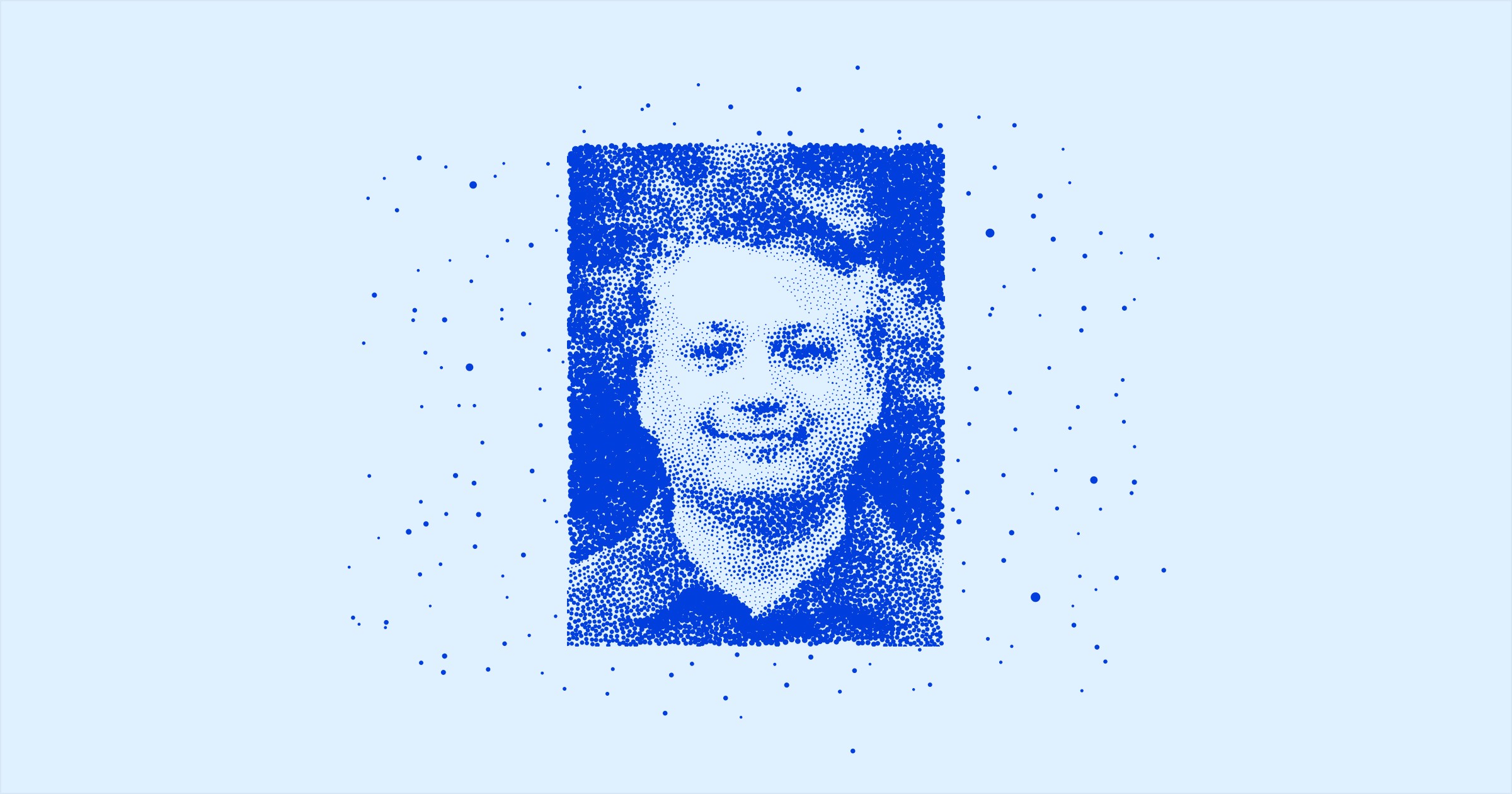
We Welcome Sam Partovi as Our Chief Commercial Officer
We Welcome Sam Partovi as Our Chief Commercial Officer
We Welcome Sam Partovi as Our Chief Commercial Officer
Sep 25, 2024
Sep 25, 2024


Affordable High-Throughput Protein Purification
Affordable High-Throughput Protein Purification
Affordable High-Throughput Protein Purification
Jul 30, 2024
Jul 30, 2024

Stay in the loop
Stay in the loop
Stay in the loop
Get new posts and other Cradle updates directly to your inbox. No spam :)