Case study: design better P450s 4X faster by combining AI-generated mutations with human expertise
Case study: design better P450s 4X faster by combining AI-generated mutations with human expertise
Case study: design better P450s 4X faster by combining AI-generated mutations with human expertise
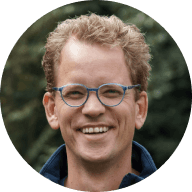
Stef van Grieken
Stef van Grieken
March 14, 2024
March 14, 2024



Customer overview
Number of Employees: 50-200
Industry: Biotechnology Research
Corporate HQ: USA
The Company harnesses the power of organisms to produce complex natural components essential to our everyday experiences, such as flavors, scents, food additives, beauty products, vitamins, medications, and agricultural agents. Through cutting-edge fermentation techniques, the Company mimics nature's processes for industrial biomanufacturing, offering eco-friendly and economically viable ingredient solutions for enhancing health, well-being, and nutrition.
Background
The Company set out on a quest to advance the biosynthesis of an active pharmaceutical ingredient. Their engineering goal was to increase the activity of a key P450 enzyme. Over 10 experimental rounds, bioengineers at the Company tested 1200 enzyme variants. The design of these variants combined a blend of cutting-edge bioinformatics, molecular modeling, and rational design techniques. To keep investing in enzyme engineering, bioengineers at the Company required a significant improvement in every round. Seeking to make the most of their project data, they partnered with Cradle to leverage custom-trained generative Machine Learning models.
For Cradle, this was one of the test cases during the earliest phases of developing its technology.
Highlights

Boosting enzymatic activity
After the Company initially completed ten rounds of mutagenesis, showcasing steady progress, Cradle's technology elevated the game by boosting substrate conversion (nearly tripling in 3 cycles) with a 4X rate of improvement over time.

Unveiling novel mutations
Using the Cradle Platform, several impactful novel mutations were unearthed. This novelty paved the way for enhanced variants and kept the door open for future diversification strategies in protein design, steering clear of potential dead-ends.

Best of both worlds
The top 5 performers from the three collaborative protein optimization rounds included either mutations suggested by Cradle, or a combination of Cradle’s and the Company's mutations. Cradle's Machine Learning platform learns from and merges various methodologies, resulting in an optimal blend of human expertise and artificial intelligence.
Project challenges
Navigating diminishing returns
As optimization rounds progress, improving enzymes becomes increasingly challenging. This diminishing return stems from several factors. The most obvious beneficial mutations have already been tested, the risk of entering a dead-end optimization path has increased, and the native folding may not accommodate additional improving mutations without compromising other characteristics. Diminished outcomes can hinder meeting industrial standards, leading to months of effort and millions of dollars invested in enzyme engineering going to waste.
Limitations of traditional approaches
The efficacy of conventional physics- and structure-based models, such as Rosetta and docking, was limited in this campaign due to the poor quality of the predicted structural model. In fact, the absence of closely related homologs with established experimental structural models (obtained through methods like X-ray diffraction, cryoEM, or NMR) constrained the predictive capability of AlphaFold.
Features of the collaboration
Retain full IP control
Ensuring the protection of Intellectual Property (IP) ranks as a primary concern for biotechnology and pharma companies. With the utilization of the Cradle platform, the Company did not need to disclose the chemical structures of substrate or product. Notably, this could have posed an insurmountable obstacle for structure-based and other state-of-the-art methods. Furthermore, the Company maintained complete IP ownership of sequences generated through Cradle. Data security was ensured through highly secure cloud machines equipped with state-of-the-art authentication. As for the models fine-tuned on the Company data, they remained, remain, and will remain inaccessible to third parties, further reinforcing data protection measures.
Leverage historical data
Prior to using the Cradle Platform, the Company's team had already tested 10 rounds of P450 variants, employing a mix of rational design and cutting-edge bioinformatics. The selected P450, which became the starting point for partnership with Cradle, showcased an more than 10-fold improvement in activity compared to the wild type. Leveraging the Company's historical data, Cradle's models were able to construct a local fitness landscape and propose novel mutations to further increase enzymatic activity. This enabled the Company's team to not only advance their previous efforts, but also to research unexplored mutations without needing to start from scratch.
Small experimental rounds
For every iteration utilizing the Cradle platform, a set of 96-192 sequences proved sufficient to train the project-specific AI model further.
Outcomes
Using the Cradle Platform the Company nearly tripled activity in three prediction-lab rounds, 4X faster than in previous rounds. The first two joint rounds saw activity more than double, with the activity versus round rate increasing. The final round built upon previous rounds, resulting in all tested mutants outperforming the top 50% of mutants of the second-to-last round. Taken together, Cradle significantly shortened the time and cost required to reach the project objective, helping bring novel processes to market faster.
Cradle's algorithms leveraged existing data effectively, recombining known mutations with novel AI-generated mutations. The use of sequence-based models eliminated the need to provide structural and chemical information of the protein and its substrate.
“Cradle's AI-based approaches were invaluable in enhancing our enzyme's activity. We were particularly impressed by its ability to effectively leverage our historical data, as well as the collaborative spirit the Cradle team exhibited throughout the project.”
Figure: Impact of Cradle on the project

Next steps
This marks one of Cradle's earliest demonstrations of value with an external collaborator. Since starting this project last year, Cradle’s models have been continuously improved, details of which will be shared in future case studies.. Below are some of the features that you can now expect when using the Cradle platform:
Autonomy in Protein Optimization: All steps taken in this collaboration can now be carried out using Cradle's intuitive user interface or api endpoints. Users without prior AI or coding experience can autonomously fine-tune models on their experimental data and run iterative optimization rounds using a user-friendly UI.
Multi-Property Approach: While this project focused solely on activity optimization, Cradle can now optimize for multiple properties concurrently, such as activity, affinity, specificity, expression, and thermostability.
Property Value Prediction: In this project, designed mutants were ranked based on performance. Today, Cradle’s Platform can predict the actual value of each target property. This advancement will inform project decisions as well as enabling accurate estimations of time to target completion.
If you are interested in accelerating your protein engineering campaigns with AI, request an invite to Cradle’s platform here.
Customer overview
Number of Employees: 50-200
Industry: Biotechnology Research
Corporate HQ: USA
The Company harnesses the power of organisms to produce complex natural components essential to our everyday experiences, such as flavors, scents, food additives, beauty products, vitamins, medications, and agricultural agents. Through cutting-edge fermentation techniques, the Company mimics nature's processes for industrial biomanufacturing, offering eco-friendly and economically viable ingredient solutions for enhancing health, well-being, and nutrition.
Background
The Company set out on a quest to advance the biosynthesis of an active pharmaceutical ingredient. Their engineering goal was to increase the activity of a key P450 enzyme. Over 10 experimental rounds, bioengineers at the Company tested 1200 enzyme variants. The design of these variants combined a blend of cutting-edge bioinformatics, molecular modeling, and rational design techniques. To keep investing in enzyme engineering, bioengineers at the Company required a significant improvement in every round. Seeking to make the most of their project data, they partnered with Cradle to leverage custom-trained generative Machine Learning models.
For Cradle, this was one of the test cases during the earliest phases of developing its technology.
Highlights

Boosting enzymatic activity
After the Company initially completed ten rounds of mutagenesis, showcasing steady progress, Cradle's technology elevated the game by boosting substrate conversion (nearly tripling in 3 cycles) with a 4X rate of improvement over time.

Unveiling novel mutations
Using the Cradle Platform, several impactful novel mutations were unearthed. This novelty paved the way for enhanced variants and kept the door open for future diversification strategies in protein design, steering clear of potential dead-ends.

Best of both worlds
The top 5 performers from the three collaborative protein optimization rounds included either mutations suggested by Cradle, or a combination of Cradle’s and the Company's mutations. Cradle's Machine Learning platform learns from and merges various methodologies, resulting in an optimal blend of human expertise and artificial intelligence.
Project challenges
Navigating diminishing returns
As optimization rounds progress, improving enzymes becomes increasingly challenging. This diminishing return stems from several factors. The most obvious beneficial mutations have already been tested, the risk of entering a dead-end optimization path has increased, and the native folding may not accommodate additional improving mutations without compromising other characteristics. Diminished outcomes can hinder meeting industrial standards, leading to months of effort and millions of dollars invested in enzyme engineering going to waste.
Limitations of traditional approaches
The efficacy of conventional physics- and structure-based models, such as Rosetta and docking, was limited in this campaign due to the poor quality of the predicted structural model. In fact, the absence of closely related homologs with established experimental structural models (obtained through methods like X-ray diffraction, cryoEM, or NMR) constrained the predictive capability of AlphaFold.
Features of the collaboration
Retain full IP control
Ensuring the protection of Intellectual Property (IP) ranks as a primary concern for biotechnology and pharma companies. With the utilization of the Cradle platform, the Company did not need to disclose the chemical structures of substrate or product. Notably, this could have posed an insurmountable obstacle for structure-based and other state-of-the-art methods. Furthermore, the Company maintained complete IP ownership of sequences generated through Cradle. Data security was ensured through highly secure cloud machines equipped with state-of-the-art authentication. As for the models fine-tuned on the Company data, they remained, remain, and will remain inaccessible to third parties, further reinforcing data protection measures.
Leverage historical data
Prior to using the Cradle Platform, the Company's team had already tested 10 rounds of P450 variants, employing a mix of rational design and cutting-edge bioinformatics. The selected P450, which became the starting point for partnership with Cradle, showcased an more than 10-fold improvement in activity compared to the wild type. Leveraging the Company's historical data, Cradle's models were able to construct a local fitness landscape and propose novel mutations to further increase enzymatic activity. This enabled the Company's team to not only advance their previous efforts, but also to research unexplored mutations without needing to start from scratch.
Small experimental rounds
For every iteration utilizing the Cradle platform, a set of 96-192 sequences proved sufficient to train the project-specific AI model further.
Outcomes
Using the Cradle Platform the Company nearly tripled activity in three prediction-lab rounds, 4X faster than in previous rounds. The first two joint rounds saw activity more than double, with the activity versus round rate increasing. The final round built upon previous rounds, resulting in all tested mutants outperforming the top 50% of mutants of the second-to-last round. Taken together, Cradle significantly shortened the time and cost required to reach the project objective, helping bring novel processes to market faster.
Cradle's algorithms leveraged existing data effectively, recombining known mutations with novel AI-generated mutations. The use of sequence-based models eliminated the need to provide structural and chemical information of the protein and its substrate.
“Cradle's AI-based approaches were invaluable in enhancing our enzyme's activity. We were particularly impressed by its ability to effectively leverage our historical data, as well as the collaborative spirit the Cradle team exhibited throughout the project.”
Figure: Impact of Cradle on the project

Next steps
This marks one of Cradle's earliest demonstrations of value with an external collaborator. Since starting this project last year, Cradle’s models have been continuously improved, details of which will be shared in future case studies.. Below are some of the features that you can now expect when using the Cradle platform:
Autonomy in Protein Optimization: All steps taken in this collaboration can now be carried out using Cradle's intuitive user interface or api endpoints. Users without prior AI or coding experience can autonomously fine-tune models on their experimental data and run iterative optimization rounds using a user-friendly UI.
Multi-Property Approach: While this project focused solely on activity optimization, Cradle can now optimize for multiple properties concurrently, such as activity, affinity, specificity, expression, and thermostability.
Property Value Prediction: In this project, designed mutants were ranked based on performance. Today, Cradle’s Platform can predict the actual value of each target property. This advancement will inform project decisions as well as enabling accurate estimations of time to target completion.
If you are interested in accelerating your protein engineering campaigns with AI, request an invite to Cradle’s platform here.


8x improvement in EGFR binding affinity: winning the Adaptyv Bio protein design competition
8x improvement in EGFR binding affinity: winning the Adaptyv Bio protein design competition
8x improvement in EGFR binding affinity: winning the Adaptyv Bio protein design competition
Dec 10, 2024
Dec 10, 2024
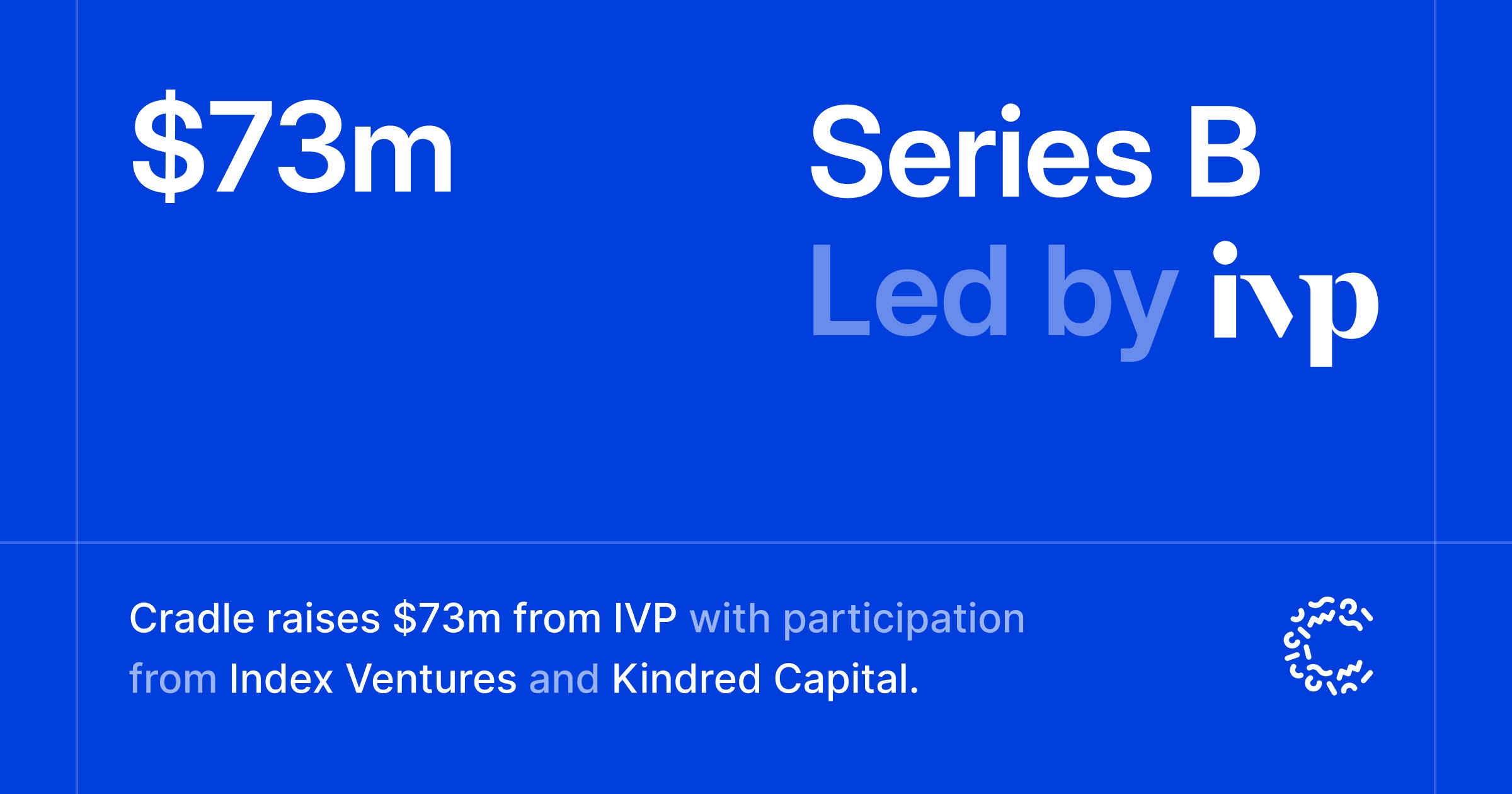
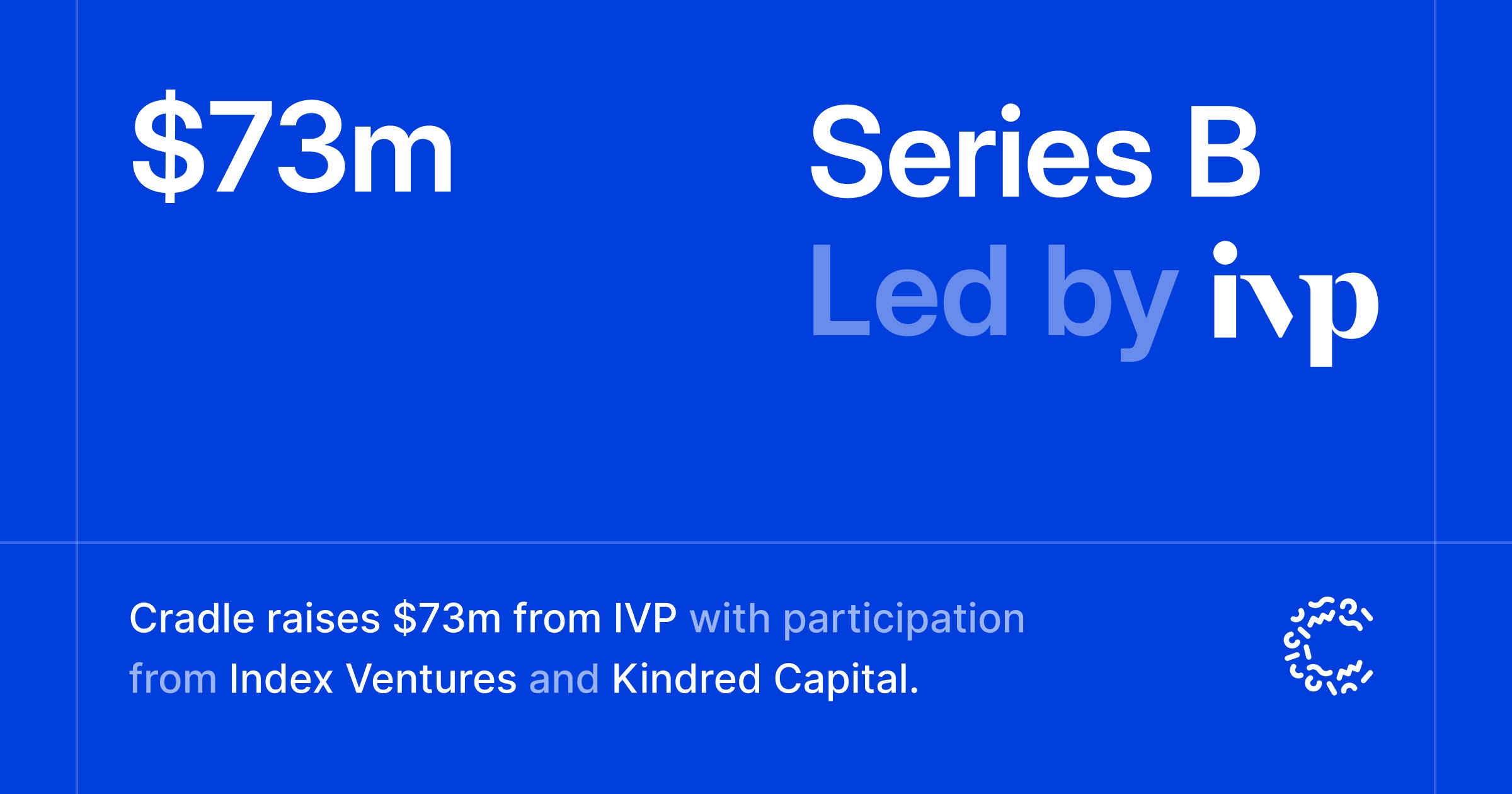
Cradle raises $73M Series B to Put AI-Powered Protein Engineering in Every Lab
Cradle raises $73M Series B to Put AI-Powered Protein Engineering in Every Lab
Cradle raises $73M Series B to Put AI-Powered Protein Engineering in Every Lab
Nov 26, 2024
Nov 26, 2024
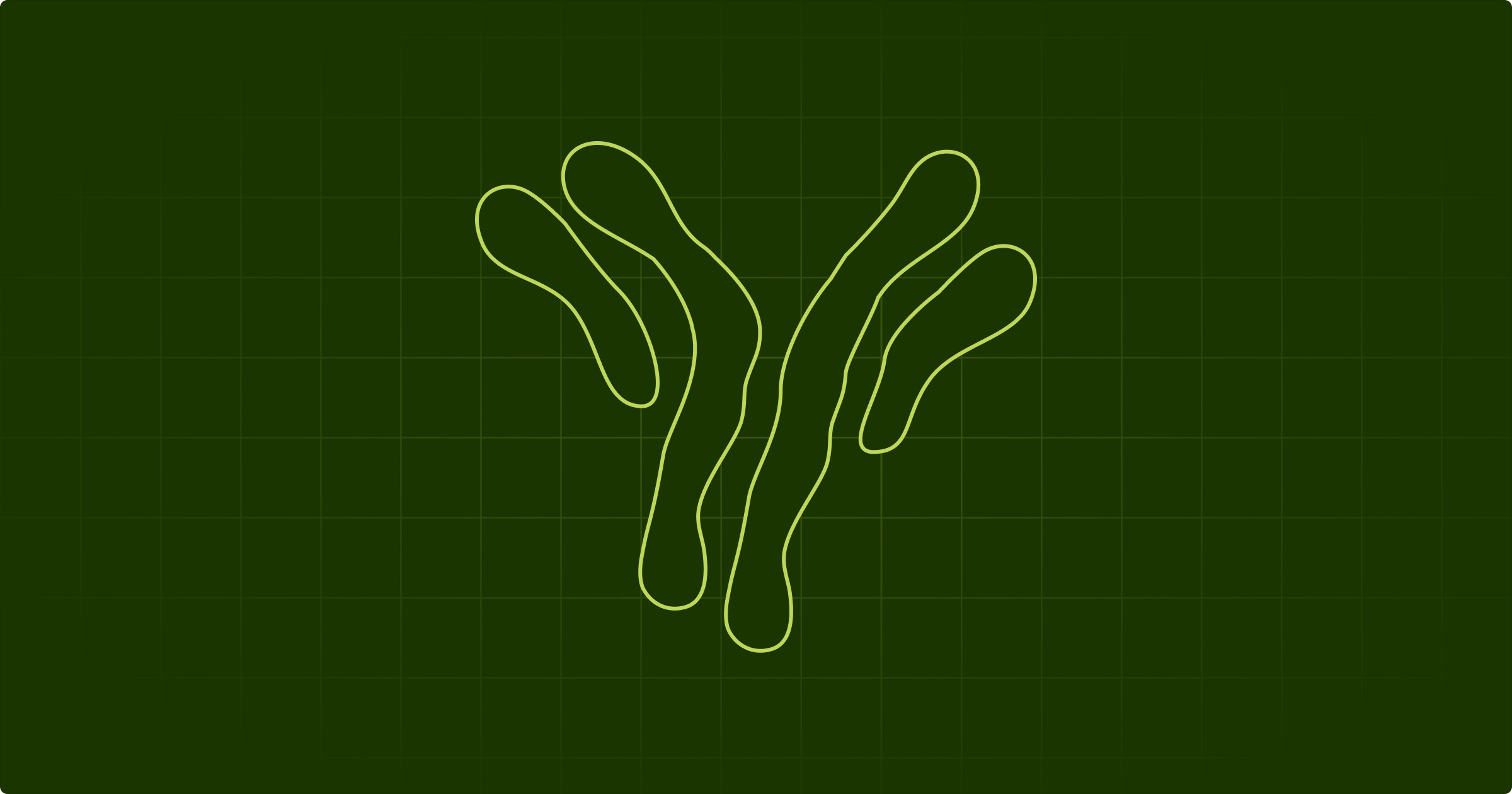
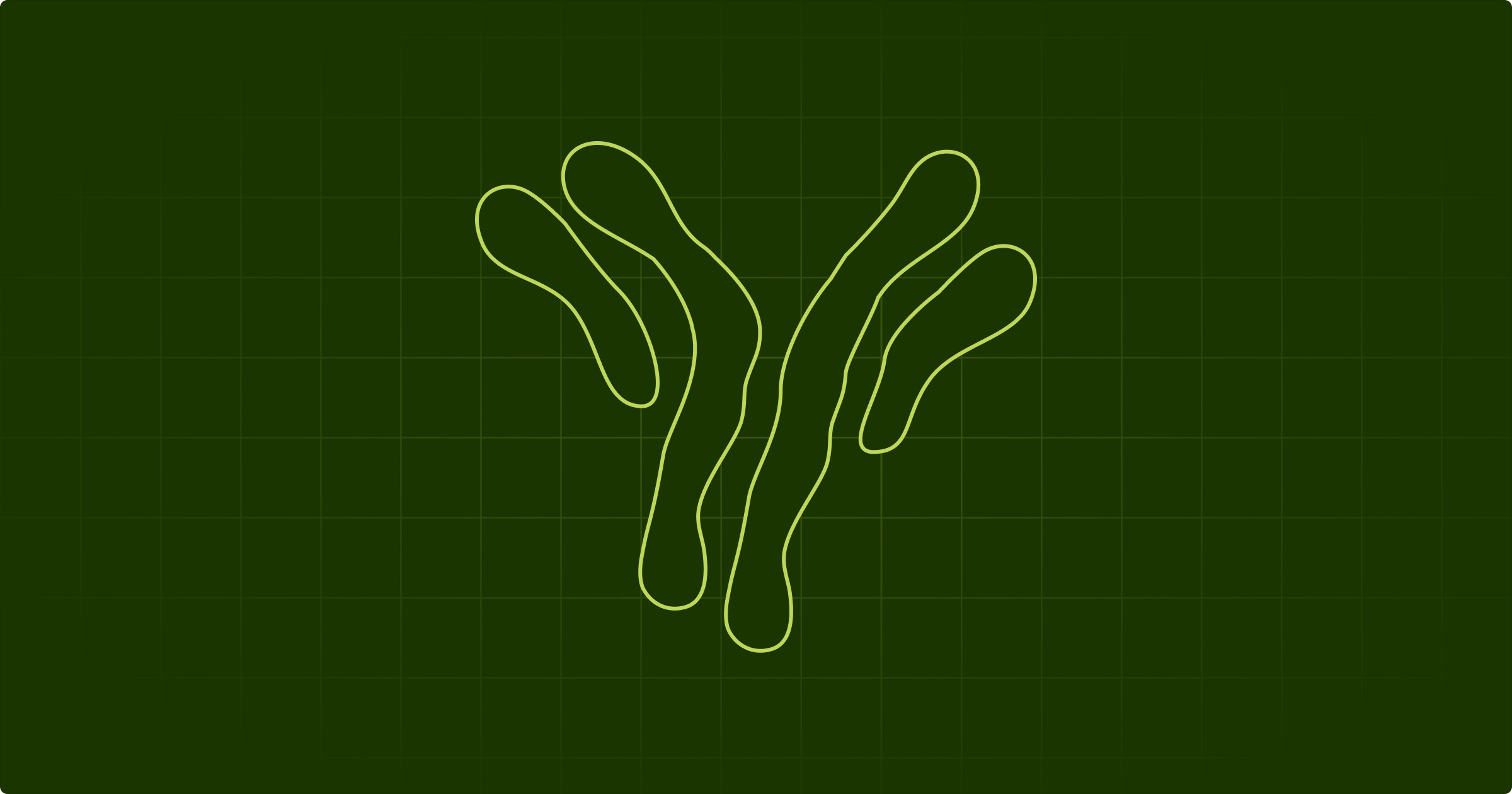
We're Funding the Creation of an Open-Source Antibody Dataset
We're Funding the Creation of an Open-Source Antibody Dataset
We're Funding the Creation of an Open-Source Antibody Dataset
Nov 11, 2024
Nov 11, 2024
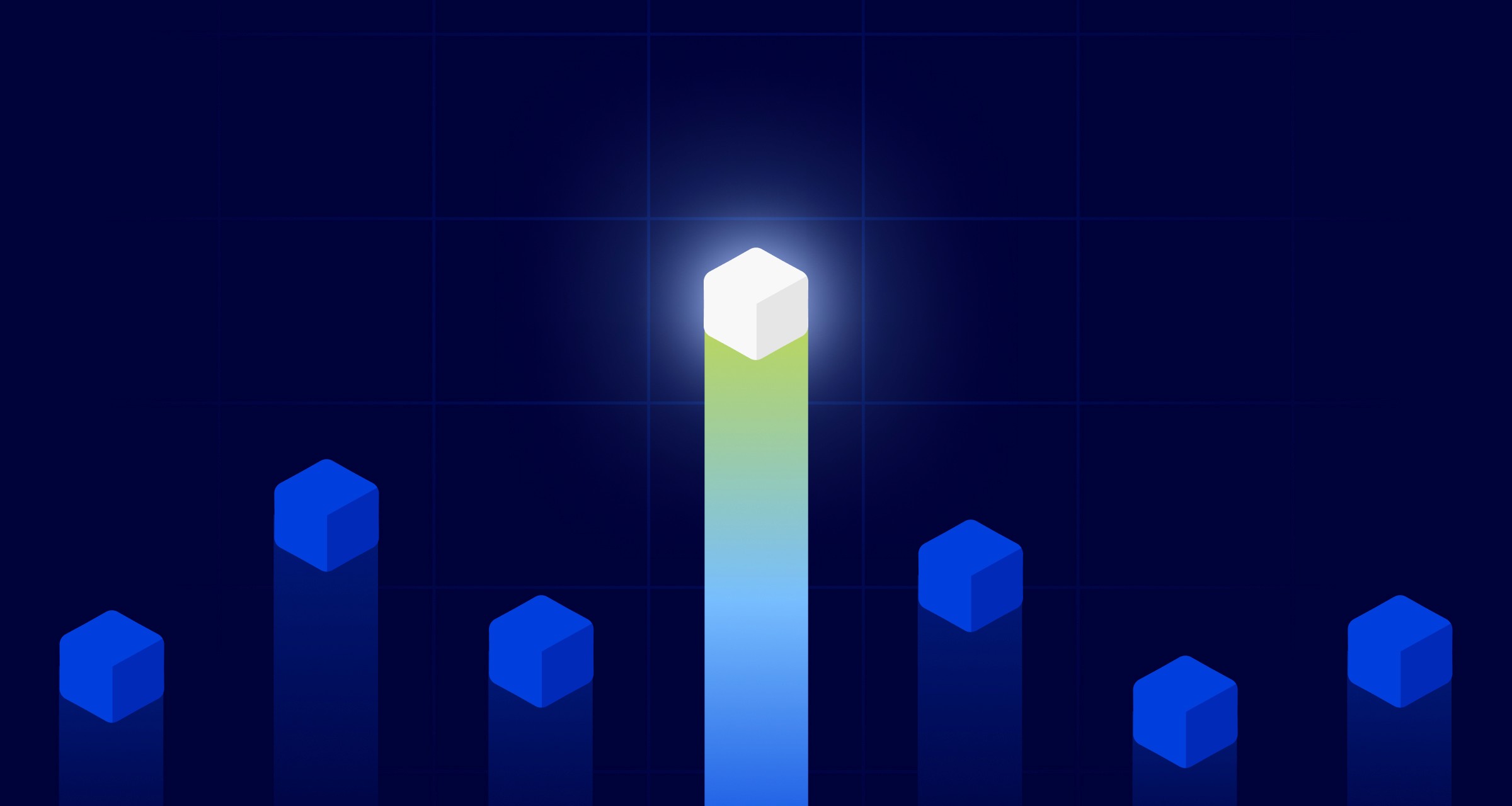
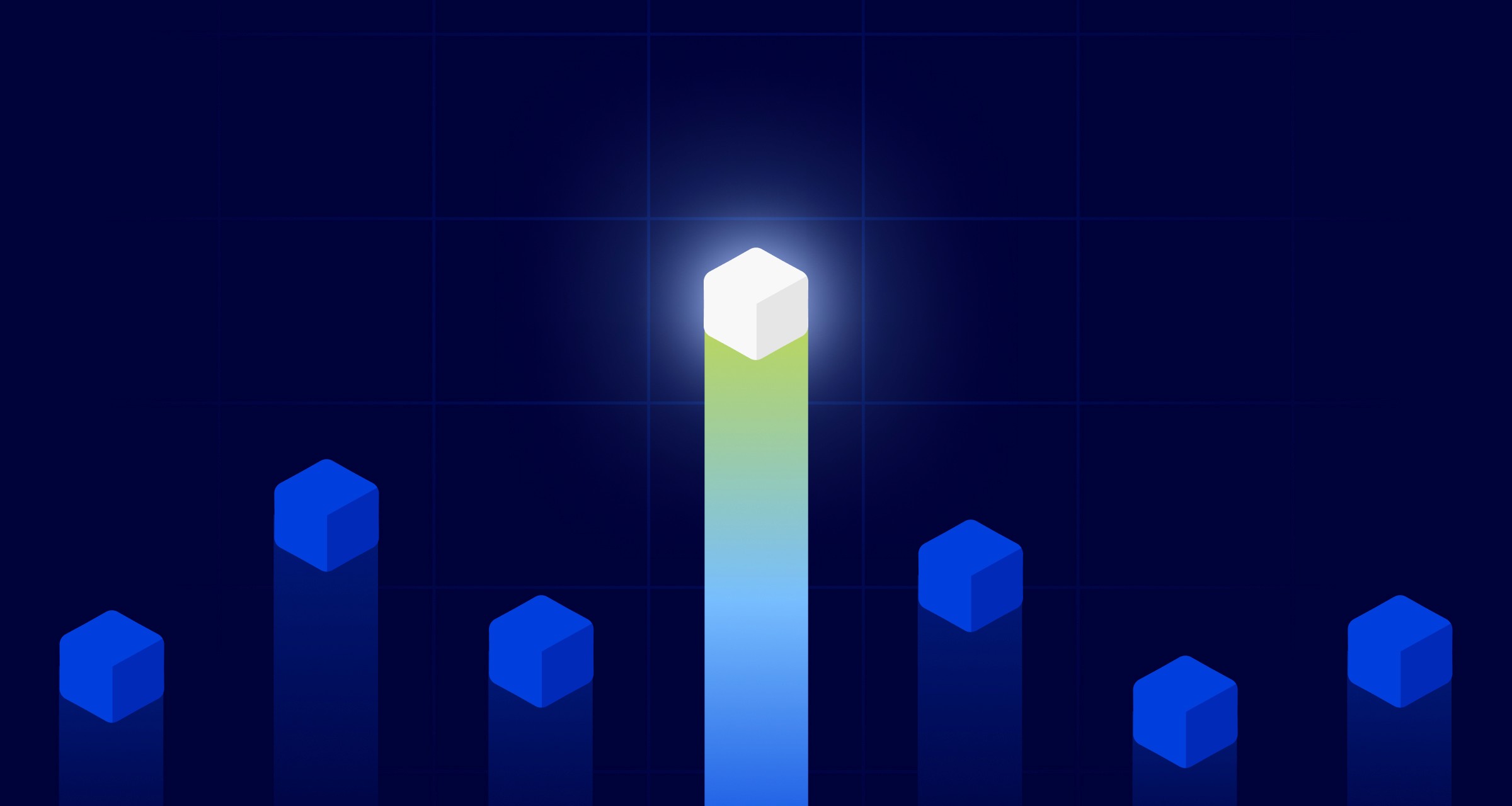
'Align to Innovate' benchmark: state-of-the-art enzyme engineering with fully-automated GenAI
'Align to Innovate' benchmark: state-of-the-art enzyme engineering with fully-automated GenAI
'Align to Innovate' benchmark: state-of-the-art enzyme engineering with fully-automated GenAI
Oct 3, 2024
Oct 3, 2024
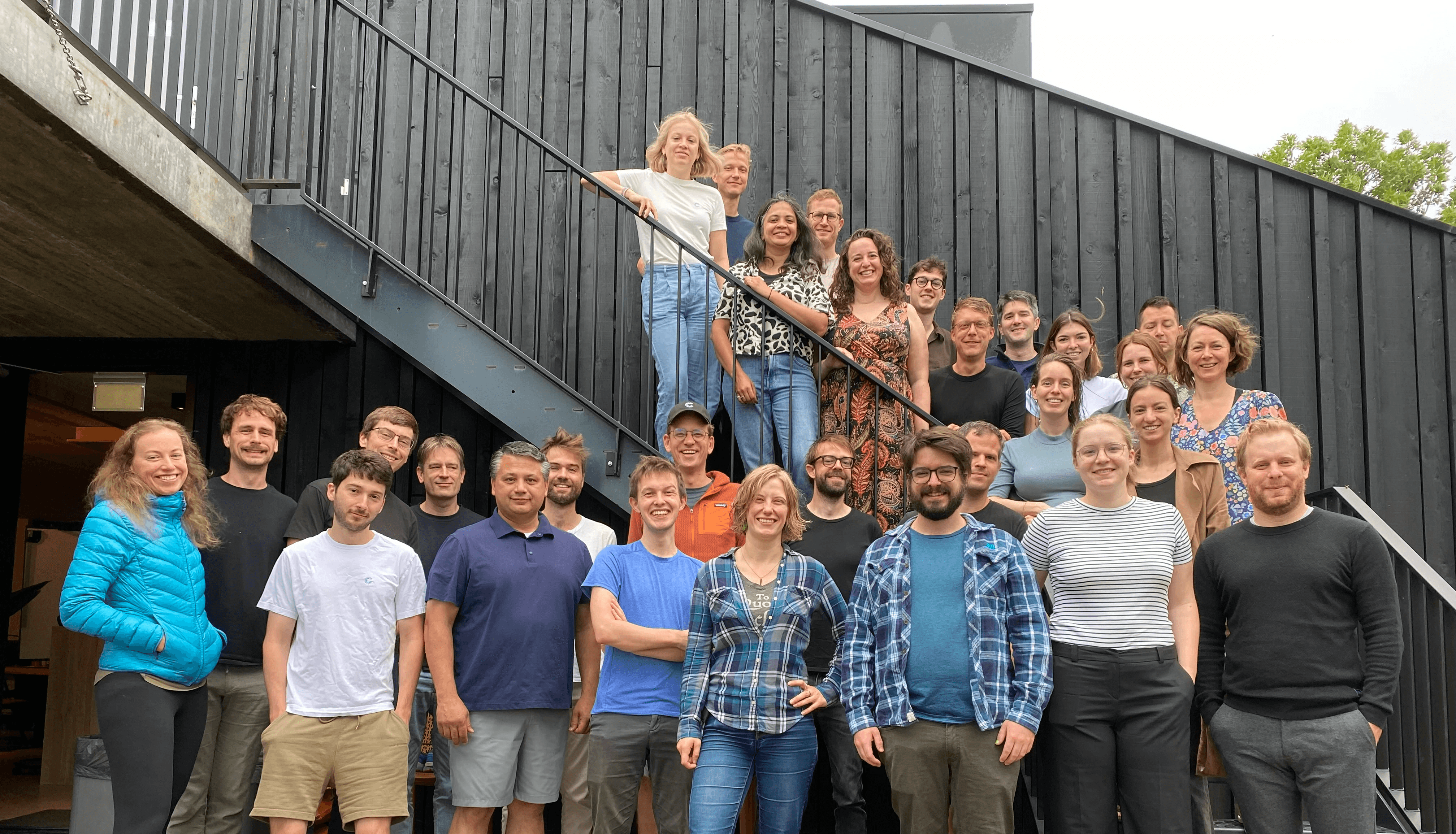
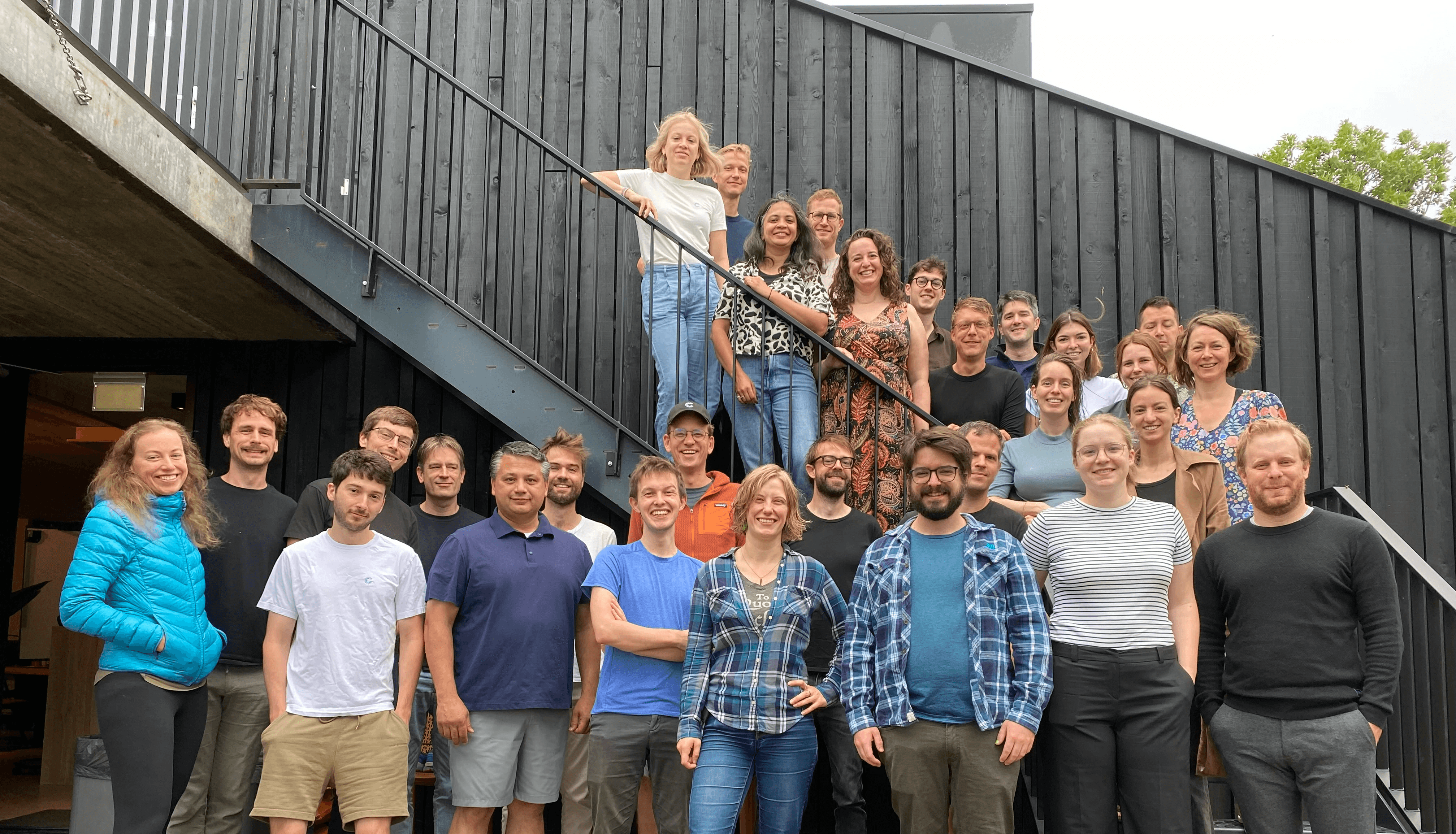
Cultural values at Cradle
Cultural values at Cradle
Cultural values at Cradle
Oct 2, 2024
Oct 2, 2024

Stay in the loop
Stay in the loop
Stay in the loop
Get new posts and other Cradle updates directly to your inbox. No spam :)