From Bench to Bytes: Why This Protein Engineer Joined an AI Startup, an Interview with Jinel Shah
From Bench to Bytes: Why This Protein Engineer Joined an AI Startup, an Interview with Jinel Shah
From Bench to Bytes: Why This Protein Engineer Joined an AI Startup, an Interview with Jinel Shah

Jelle Prins
Jelle Prins
June 5, 2024
June 5, 2024
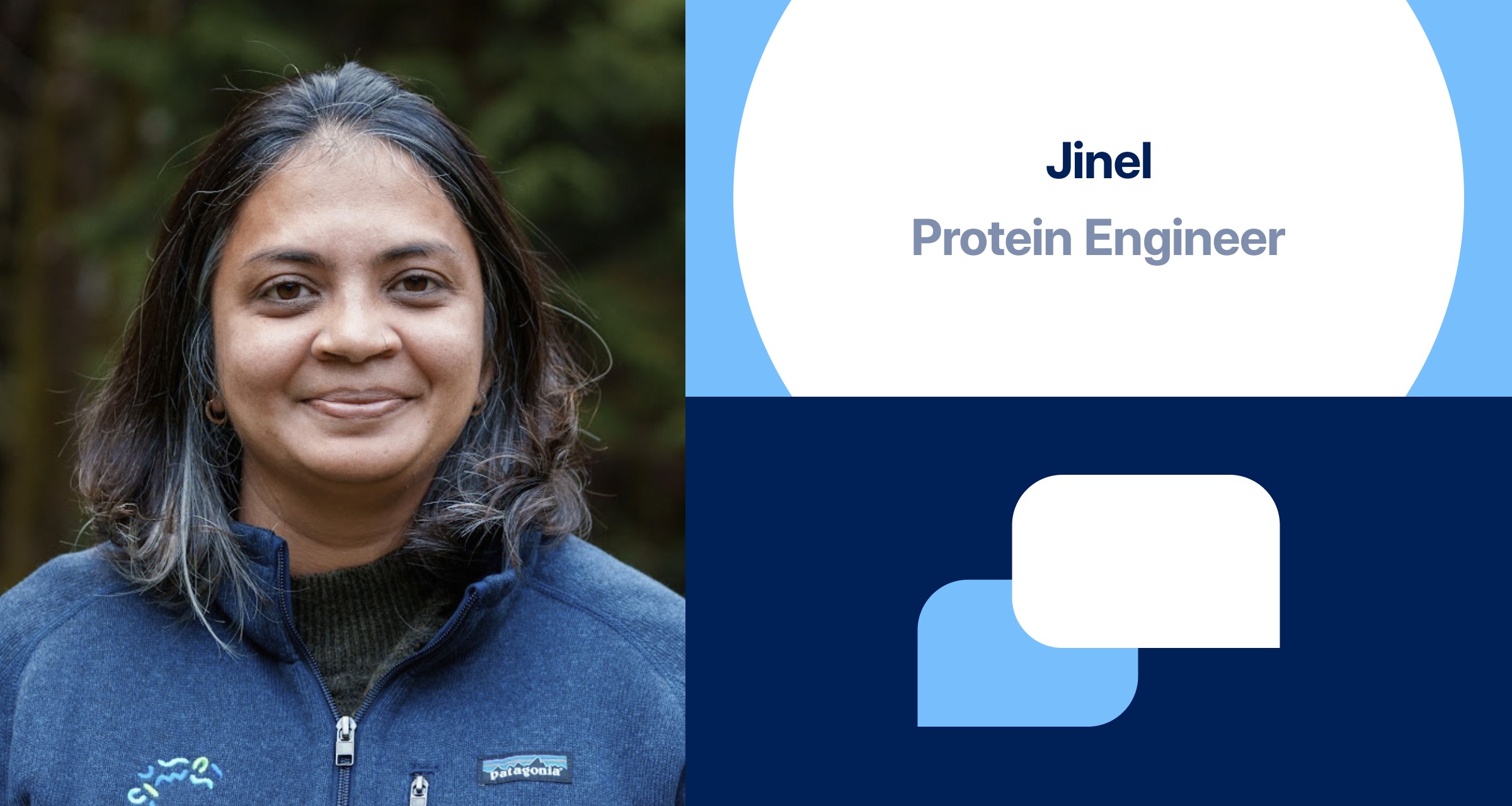
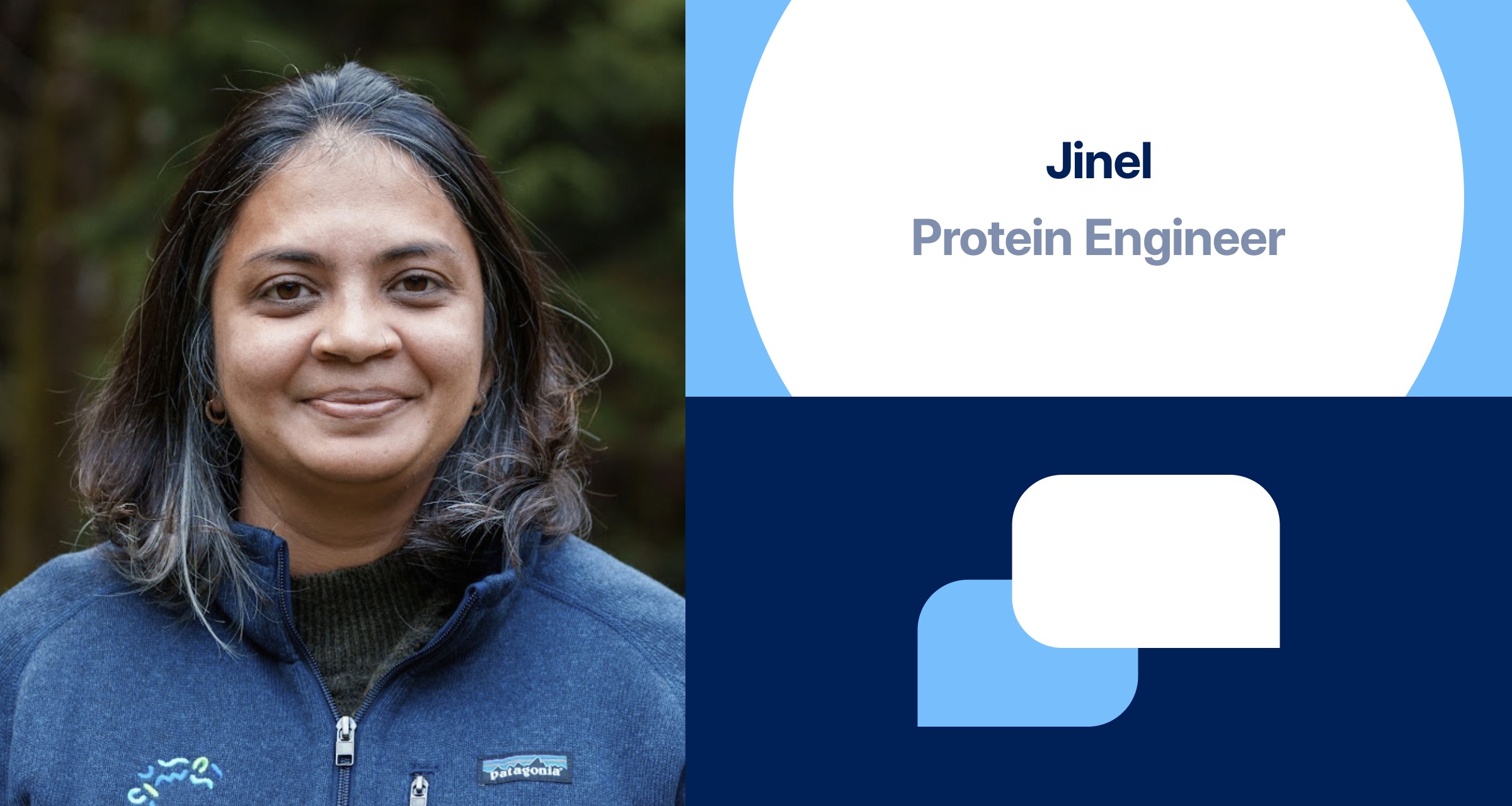
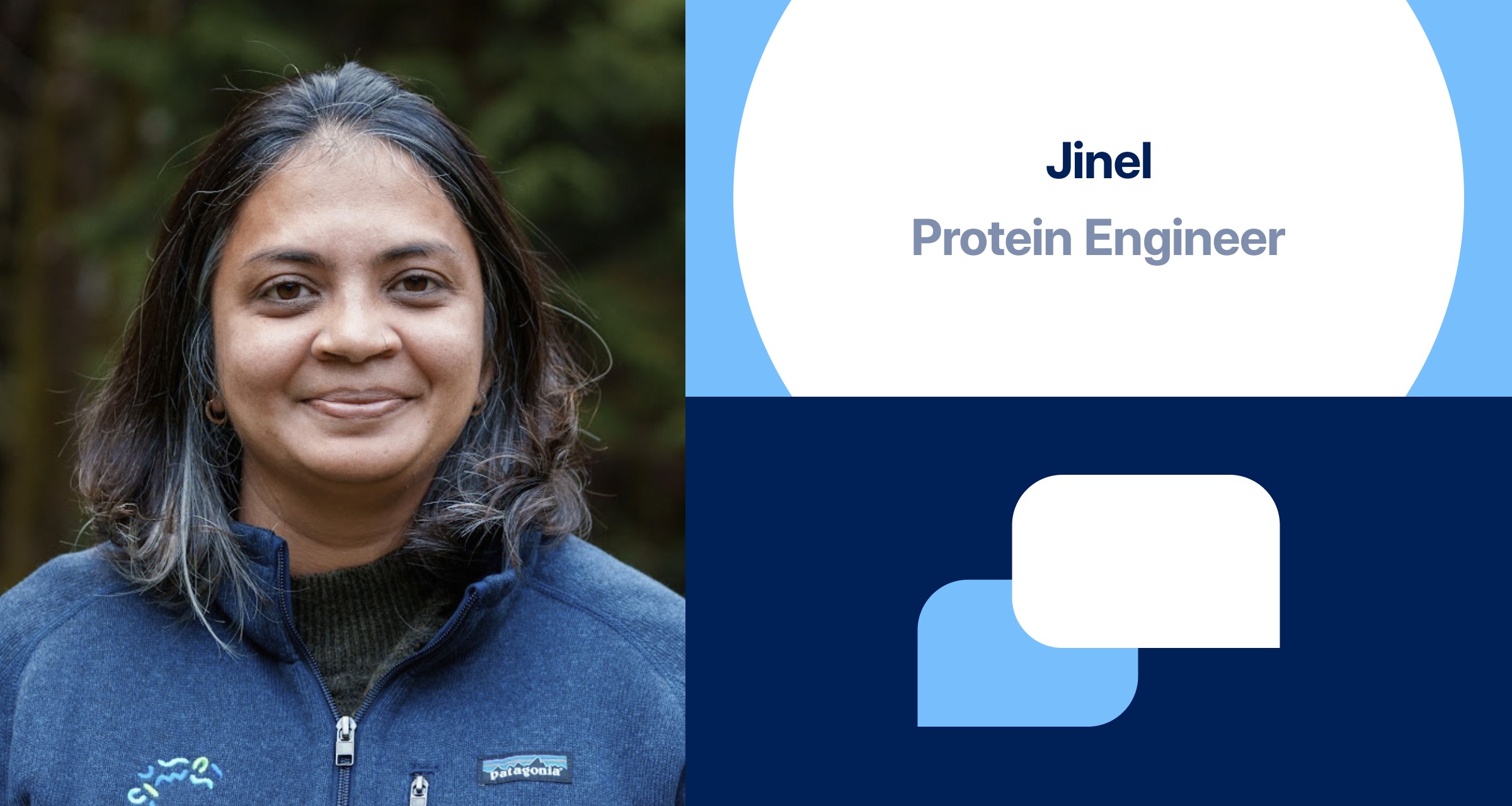
Welcome to a new Cradle Team Spotlight, highlighting the inner workings of Cradle and the people behind the technology.
Our second feature is Jinel Shah, one of the scientists working on streamlining Cradle’s wet lab operations. In this conversation, Jinel shares her thoughts on the ‘human vs machine’ battle, the biggest bottlenecks in protein engineering, and the importance of bridging the gap between biologists and mathematicians.
‘Like thousands of bioinformaticians working together’
In a way, Jinel represents the archetypal user of Cradle’s platform. Before joining Cradle, she was a protein engineer at Genomatica for almost 8 years. There, Jinel worked on a multi-year project to commercialize the production of Genomatica’s plant-based nylon, which involved improving several key proteins. More often than not, however, she would catch herself wishing she had better protein engineering tools.
“Protein engineers like me, we spend a lot of our time looking at protein structures, one by one, and trying to figure out what mutations could improve it,” explains Jinel.
Most biologists don’t have the time to learn coding. So, they work with bioinformaticians to look at dozens of bacterial protein sequences, searching for clues on how to make their protein better. Then, they generate and test thousands of variants in the lab. This process often takes months, or even years in some cases.
“Even if I knew how to code, I would still be just one bioinformatician doing my own analysis,” says Jinel. “Whereas a machine learning model is trained on billions of sequences and can figure out which amino acid change will provide the biggest benefit. It’s like thousands of bioinformaticians working together.”
“You are learning from every single result, even the failures. You are teaching the model the rules of protein design.”
To give an example, one of the proteins Jinel engineered had ten beneficial mutations accumulated over ten rounds of engineering. This project took several years to carry out, mimicking the step-by-step process of natural evolution where changes happen one mutation at a time. Only the beneficial mutations are selected and carried forward. Each new mutation has to work well with the other ones, and if it does not, that means taking a step back to find other combinations that might work better. It’s a lot of trial and error.
In contrast, the designs proposed by Cradle’s machine learning models can easily introduce 4-5 or more mutations in a single round. This offers a bigger step change and, more importantly, enables the exploration of which mutations work well together and which ones do not:
“The model learns not only from the positive but also from the negative results,” says Jinel. “It remembers which combinations don’t work well together, so it will try different ones next time. What’s more, not only does it remember which mutations work or do not work well together, but, like some sort of protein engineer prodigy, it figures out the effect of combinations it has never seen before.”
“This way you are not just learning from the positive results you usually collect,” emphasizes Jinel. “You are learning from every single result, even the failures. You are teaching the model the rules of protein design.”

De-bottlenecking protein engineering
With so much experience behind her back, Jinel recognized that machine learning was the “next step in protein engineering” and decided to join the protein design revolution. At Cradle, she develops enzyme activity assays and helps streamline high-throughput laboratory workflows.
The ‘pilot project’ for Cradle’s design platform was testing the capability of AI to improve protein stability. Now, Cradle works on multi-property optimization projects, such as improving the thermostability, increasing activity, or refining specificity all at once.
When a partner comes to Cradle, the models are trained to address their specific challenges. Over multiple rounds of iterative testing, the models get progressively better at making protein design decisions. Cradle has a variety of internal projects to provide a constant feedback loop for the ML engineers. Whenever we make changes to the models, we want to test and see if they provide actual improvements. The faster we can test these hypotheses, the quicker our models improve.
Automating and streamlining assays is Jinel’s expertise. However, she notes that developing high-throughput enzyme testing workflows takes on a slightly different form at Cradle. For example, large companies invest a lot of resources in throughput and automation to build the capacity to test hundreds of thousands of variants. Yet, increasing throughput does not solve all problems:
“At my previous company, we used to test at least twenty 384-well plates per round. With that kind of throughput, you're unable to purify so many samples, so you're doing assays in cell lysates. And that adds noise to your data,” explains Jinel. “But at Cradle, we only need to test 384 samples per round. So, we can purify them all and get very clean data because the signal is specific to that protein, not all the other potential variables within the cell.”
Cradle’s throughput comes from the machine learning aspect: “Instead of just making random mutations and doing screens after screens, we are putting more thought into what variants we are going to test in the first place,” explains Jinel. “The model has already sorted through millions of sequences, so we don’t have to do so much of the grunt work.”
What is the biggest bottleneck in the speed of protein engineering then?“At this point, our main bottleneck is biology,” says Jinel. “Cells are going to still take time to grow.”
“Instead of just making random mutations and doing screens after screens, we are putting more thought into what variants we are going to test in the first place,”

Woman vs. Machine
When Jinel joined Cradle in December 2023, her immediate first impression was: “Wow, this company is doing something really exciting”. Without previous experience in machine learning, however, the task of working for an AI protein engineering company seemed daunting: “Machine learning can seem like a black box,” confesses Jinel. “But I wanted to understand how it works.” So, she took the plunge.
After working at Cradle for a few months, Jinel realized that it was not a black box after all. An important part of mastering this new technology, she thinks, is learning to speak a different science language and being willing to ask questions: “Here at Cradle, everyone is very open. You can ask people: ‘What do you mean by this?’ Because a ‘control’ in biology is different from a ‘control’ in machine learning.”
“I used to only work with people who were experts in the same area as me,” says Jinel. “Now I’m working together with other biologists, mathematicians, designers, and computer scientists. And we have to work together because it takes that combined expertise to solve complex biology problems.”
This diversity of inputs and perspectives means we are always questioning our assumptions: not relying fully on one algorithm or one way of framing the problem but staying curious. In the spirit of that, Cradle routinely hosts a challenge to see who would be better at protein design prediction: a well-trained protein engineer or a machine learning model.
“The point of the challenge is not to see who is smarter; it’s about improving what we do,” says Jinel. “Having this immense computational power changes how scientists think about protein engineering. It encourages us to think outside the box and come up with new hypotheses.”
“I've already volunteered for the next ‘Woman vs Machine’ competition,” laughs Jinel. “I want to see how I do. I have a lot of experience so I can look at the structure and say ‘Okay, let's try these mutations.’ And that's what's exciting about Cradle: we don't think our models know best. We are open to accepting the users’ input.”
Ultimately, we believe that the best results are achieved when humans and machines work together. AI is not going to replace biologists or bioinformaticians. It’s going to help them make better decisions and be more efficient.
“Having this immense computational power changes how scientists think about protein engineering. It encourages us to think outside the box and come up with new hypotheses.”

Making machine learning for biology more accessible
When asked about what Cradle’s biggest contribution to the field is, Jinel responds: “I think Cradle is making biology and machine learning more accessible. We are not only making protein engineering easier but also helping bridge the gap between biologists and mathematicians. We are trying to change the perception that these two fields are mutually exclusive by showing that they can work together, and that will benefit everybody.”
“Even internally we are bridging that gap,” she adds. “We, the biologists, are explaining to the mathematicians that biology rules are much more fluid. For example, in mathematics, the solution to an equation does not change over time. But in biology, there are so many variables that the result you get today might be different from what you will get tomorrow, and we have to account for that uncertainty.”
Cradle’s goal is to educate more people on the power of machine learning tools for biology. A big part of that is sharing the knowledge on how to take advantage of machine learning in biology through articles, blog posts, and other means. The more people learn about this new field and get engaged, the more benefit it will have for our society at large.
“Usually, companies don't want to share their resources and learnings, but here at Cradle we’re very open about what we do and why. I think that's pretty unique,” says Jinel.
Welcome to a new Cradle Team Spotlight, highlighting the inner workings of Cradle and the people behind the technology.
Our second feature is Jinel Shah, one of the scientists working on streamlining Cradle’s wet lab operations. In this conversation, Jinel shares her thoughts on the ‘human vs machine’ battle, the biggest bottlenecks in protein engineering, and the importance of bridging the gap between biologists and mathematicians.
‘Like thousands of bioinformaticians working together’
In a way, Jinel represents the archetypal user of Cradle’s platform. Before joining Cradle, she was a protein engineer at Genomatica for almost 8 years. There, Jinel worked on a multi-year project to commercialize the production of Genomatica’s plant-based nylon, which involved improving several key proteins. More often than not, however, she would catch herself wishing she had better protein engineering tools.
“Protein engineers like me, we spend a lot of our time looking at protein structures, one by one, and trying to figure out what mutations could improve it,” explains Jinel.
Most biologists don’t have the time to learn coding. So, they work with bioinformaticians to look at dozens of bacterial protein sequences, searching for clues on how to make their protein better. Then, they generate and test thousands of variants in the lab. This process often takes months, or even years in some cases.
“Even if I knew how to code, I would still be just one bioinformatician doing my own analysis,” says Jinel. “Whereas a machine learning model is trained on billions of sequences and can figure out which amino acid change will provide the biggest benefit. It’s like thousands of bioinformaticians working together.”
“You are learning from every single result, even the failures. You are teaching the model the rules of protein design.”
To give an example, one of the proteins Jinel engineered had ten beneficial mutations accumulated over ten rounds of engineering. This project took several years to carry out, mimicking the step-by-step process of natural evolution where changes happen one mutation at a time. Only the beneficial mutations are selected and carried forward. Each new mutation has to work well with the other ones, and if it does not, that means taking a step back to find other combinations that might work better. It’s a lot of trial and error.
In contrast, the designs proposed by Cradle’s machine learning models can easily introduce 4-5 or more mutations in a single round. This offers a bigger step change and, more importantly, enables the exploration of which mutations work well together and which ones do not:
“The model learns not only from the positive but also from the negative results,” says Jinel. “It remembers which combinations don’t work well together, so it will try different ones next time. What’s more, not only does it remember which mutations work or do not work well together, but, like some sort of protein engineer prodigy, it figures out the effect of combinations it has never seen before.”
“This way you are not just learning from the positive results you usually collect,” emphasizes Jinel. “You are learning from every single result, even the failures. You are teaching the model the rules of protein design.”

De-bottlenecking protein engineering
With so much experience behind her back, Jinel recognized that machine learning was the “next step in protein engineering” and decided to join the protein design revolution. At Cradle, she develops enzyme activity assays and helps streamline high-throughput laboratory workflows.
The ‘pilot project’ for Cradle’s design platform was testing the capability of AI to improve protein stability. Now, Cradle works on multi-property optimization projects, such as improving the thermostability, increasing activity, or refining specificity all at once.
When a partner comes to Cradle, the models are trained to address their specific challenges. Over multiple rounds of iterative testing, the models get progressively better at making protein design decisions. Cradle has a variety of internal projects to provide a constant feedback loop for the ML engineers. Whenever we make changes to the models, we want to test and see if they provide actual improvements. The faster we can test these hypotheses, the quicker our models improve.
Automating and streamlining assays is Jinel’s expertise. However, she notes that developing high-throughput enzyme testing workflows takes on a slightly different form at Cradle. For example, large companies invest a lot of resources in throughput and automation to build the capacity to test hundreds of thousands of variants. Yet, increasing throughput does not solve all problems:
“At my previous company, we used to test at least twenty 384-well plates per round. With that kind of throughput, you're unable to purify so many samples, so you're doing assays in cell lysates. And that adds noise to your data,” explains Jinel. “But at Cradle, we only need to test 384 samples per round. So, we can purify them all and get very clean data because the signal is specific to that protein, not all the other potential variables within the cell.”
Cradle’s throughput comes from the machine learning aspect: “Instead of just making random mutations and doing screens after screens, we are putting more thought into what variants we are going to test in the first place,” explains Jinel. “The model has already sorted through millions of sequences, so we don’t have to do so much of the grunt work.”
What is the biggest bottleneck in the speed of protein engineering then?“At this point, our main bottleneck is biology,” says Jinel. “Cells are going to still take time to grow.”
“Instead of just making random mutations and doing screens after screens, we are putting more thought into what variants we are going to test in the first place,”

Woman vs. Machine
When Jinel joined Cradle in December 2023, her immediate first impression was: “Wow, this company is doing something really exciting”. Without previous experience in machine learning, however, the task of working for an AI protein engineering company seemed daunting: “Machine learning can seem like a black box,” confesses Jinel. “But I wanted to understand how it works.” So, she took the plunge.
After working at Cradle for a few months, Jinel realized that it was not a black box after all. An important part of mastering this new technology, she thinks, is learning to speak a different science language and being willing to ask questions: “Here at Cradle, everyone is very open. You can ask people: ‘What do you mean by this?’ Because a ‘control’ in biology is different from a ‘control’ in machine learning.”
“I used to only work with people who were experts in the same area as me,” says Jinel. “Now I’m working together with other biologists, mathematicians, designers, and computer scientists. And we have to work together because it takes that combined expertise to solve complex biology problems.”
This diversity of inputs and perspectives means we are always questioning our assumptions: not relying fully on one algorithm or one way of framing the problem but staying curious. In the spirit of that, Cradle routinely hosts a challenge to see who would be better at protein design prediction: a well-trained protein engineer or a machine learning model.
“The point of the challenge is not to see who is smarter; it’s about improving what we do,” says Jinel. “Having this immense computational power changes how scientists think about protein engineering. It encourages us to think outside the box and come up with new hypotheses.”
“I've already volunteered for the next ‘Woman vs Machine’ competition,” laughs Jinel. “I want to see how I do. I have a lot of experience so I can look at the structure and say ‘Okay, let's try these mutations.’ And that's what's exciting about Cradle: we don't think our models know best. We are open to accepting the users’ input.”
Ultimately, we believe that the best results are achieved when humans and machines work together. AI is not going to replace biologists or bioinformaticians. It’s going to help them make better decisions and be more efficient.
“Having this immense computational power changes how scientists think about protein engineering. It encourages us to think outside the box and come up with new hypotheses.”

Making machine learning for biology more accessible
When asked about what Cradle’s biggest contribution to the field is, Jinel responds: “I think Cradle is making biology and machine learning more accessible. We are not only making protein engineering easier but also helping bridge the gap between biologists and mathematicians. We are trying to change the perception that these two fields are mutually exclusive by showing that they can work together, and that will benefit everybody.”
“Even internally we are bridging that gap,” she adds. “We, the biologists, are explaining to the mathematicians that biology rules are much more fluid. For example, in mathematics, the solution to an equation does not change over time. But in biology, there are so many variables that the result you get today might be different from what you will get tomorrow, and we have to account for that uncertainty.”
Cradle’s goal is to educate more people on the power of machine learning tools for biology. A big part of that is sharing the knowledge on how to take advantage of machine learning in biology through articles, blog posts, and other means. The more people learn about this new field and get engaged, the more benefit it will have for our society at large.
“Usually, companies don't want to share their resources and learnings, but here at Cradle we’re very open about what we do and why. I think that's pretty unique,” says Jinel.


8x improvement in EGFR binding affinity: winning the Adaptyv Bio protein design competition
8x improvement in EGFR binding affinity: winning the Adaptyv Bio protein design competition
8x improvement in EGFR binding affinity: winning the Adaptyv Bio protein design competition
Dec 10, 2024
Dec 10, 2024
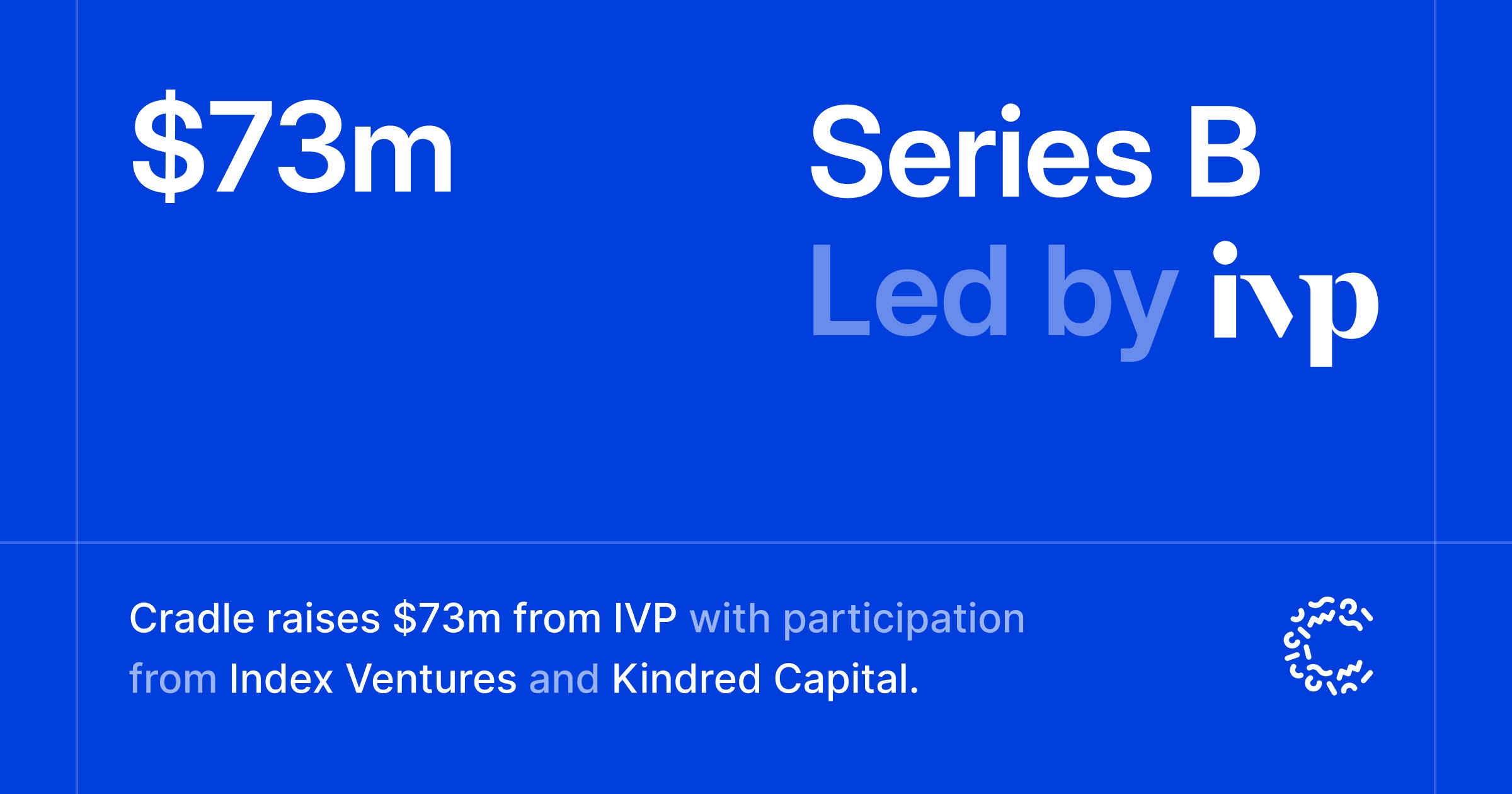
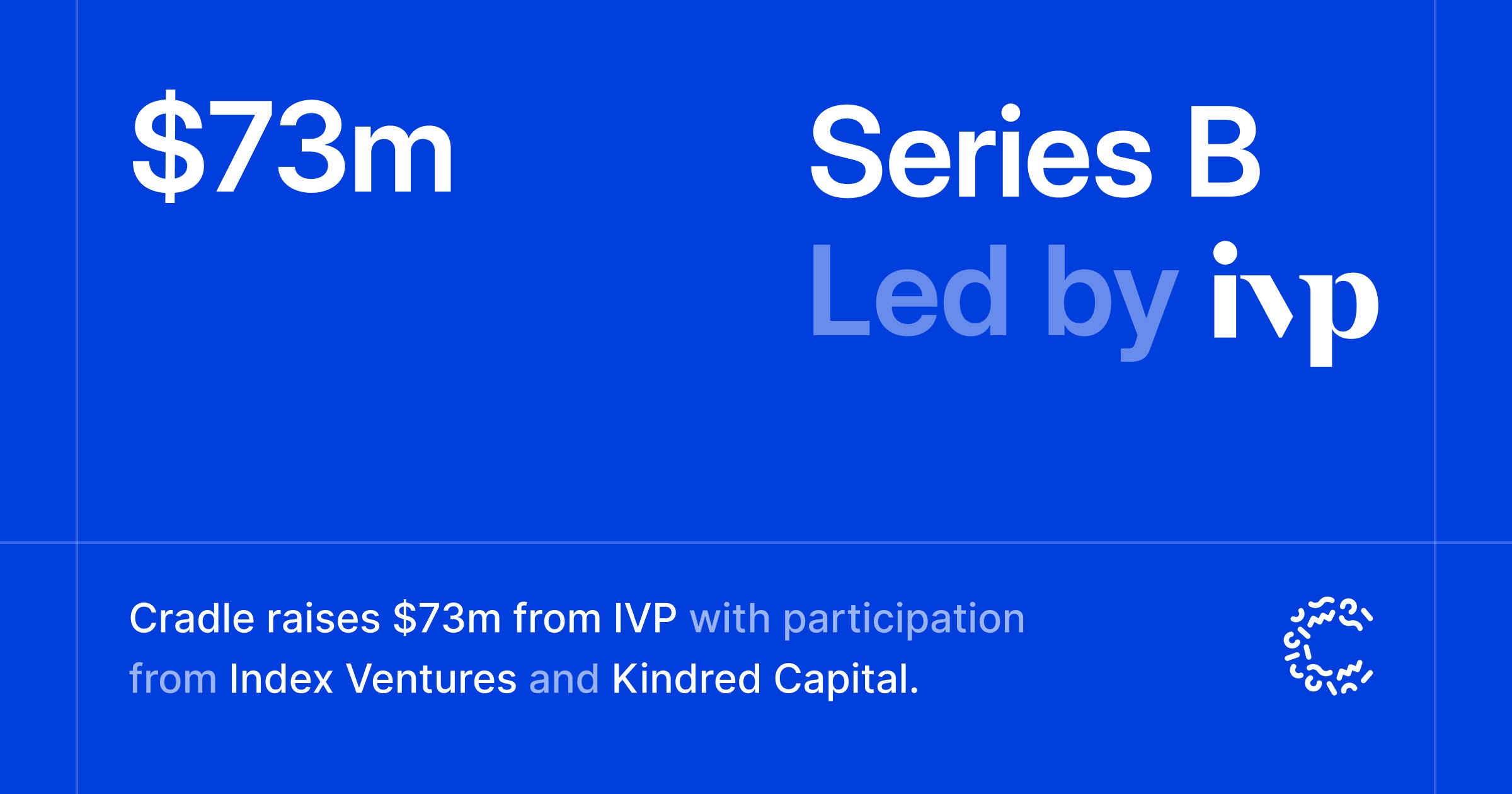
Cradle raises $73M Series B to Put AI-Powered Protein Engineering in Every Lab
Cradle raises $73M Series B to Put AI-Powered Protein Engineering in Every Lab
Cradle raises $73M Series B to Put AI-Powered Protein Engineering in Every Lab
Nov 26, 2024
Nov 26, 2024
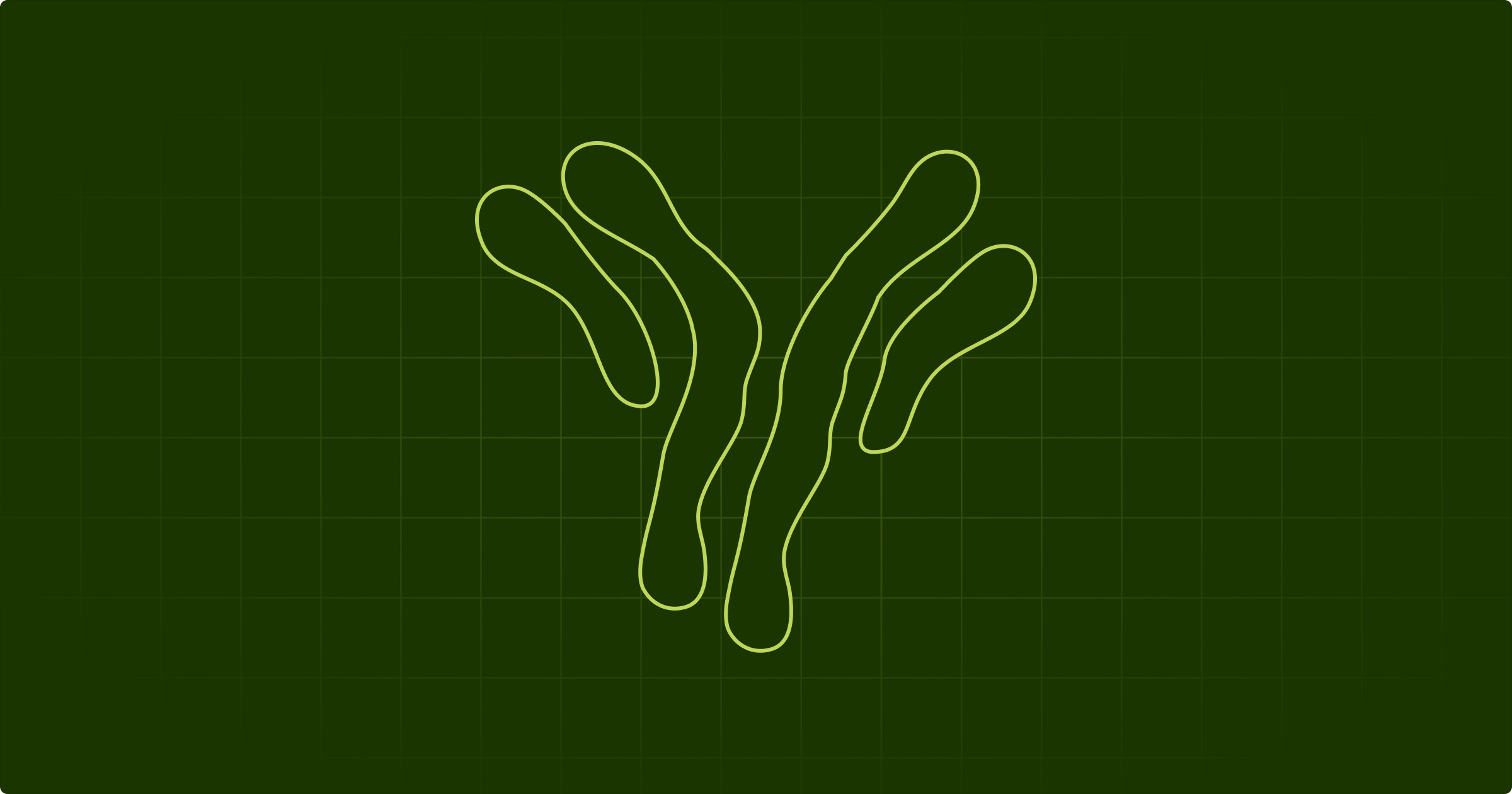
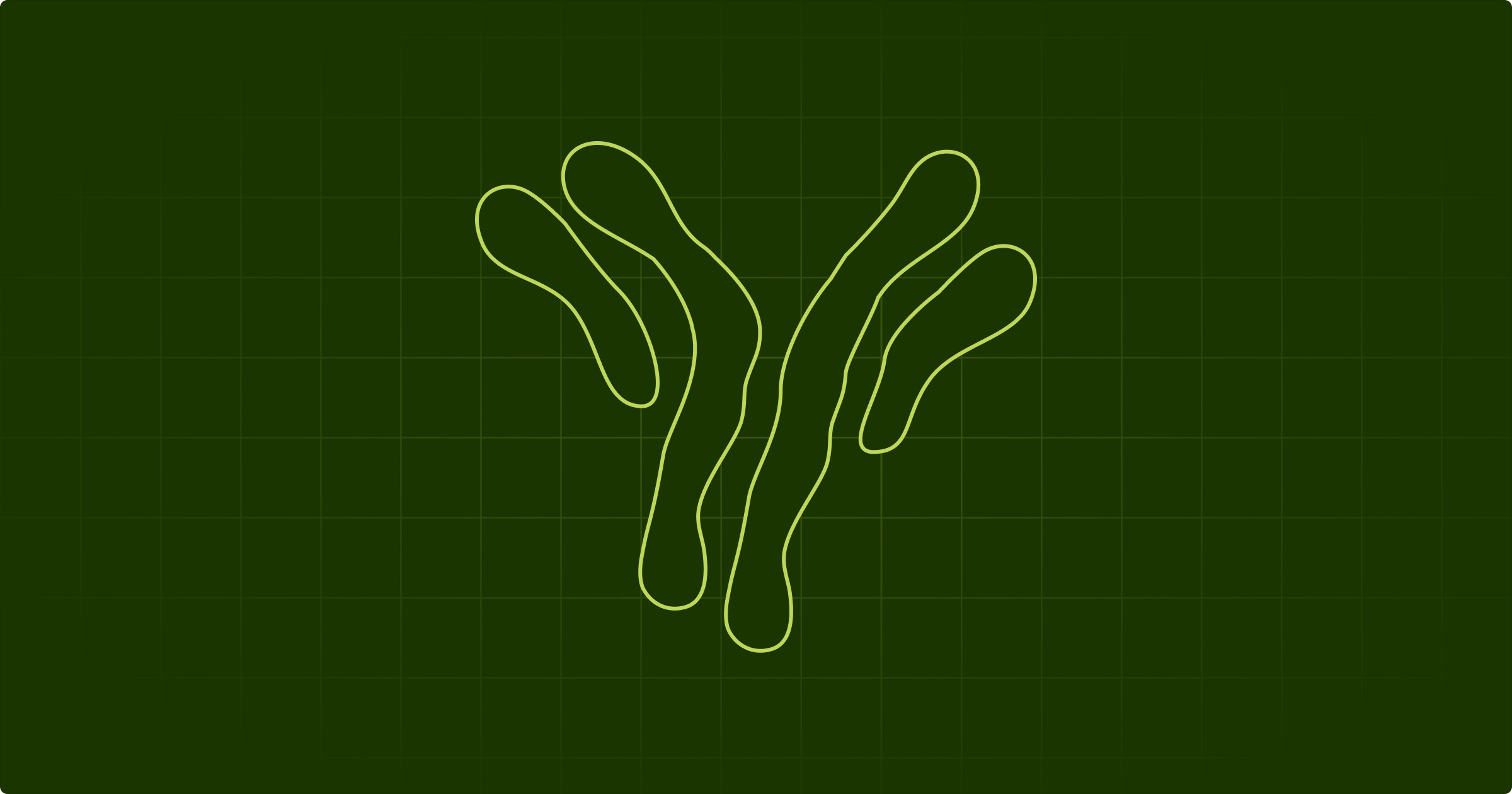
We're Funding the Creation of an Open-Source Antibody Dataset
We're Funding the Creation of an Open-Source Antibody Dataset
We're Funding the Creation of an Open-Source Antibody Dataset
Nov 11, 2024
Nov 11, 2024
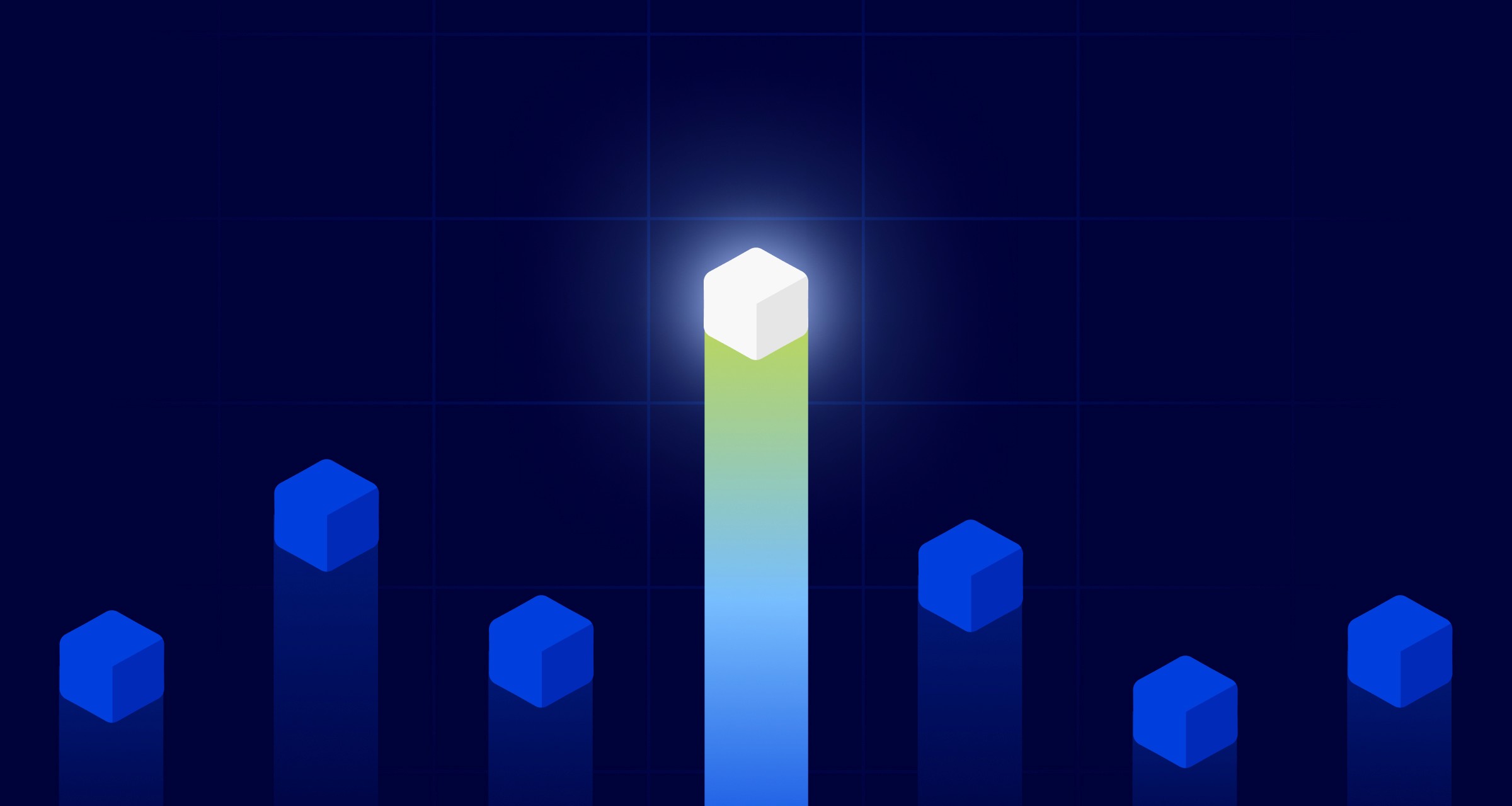
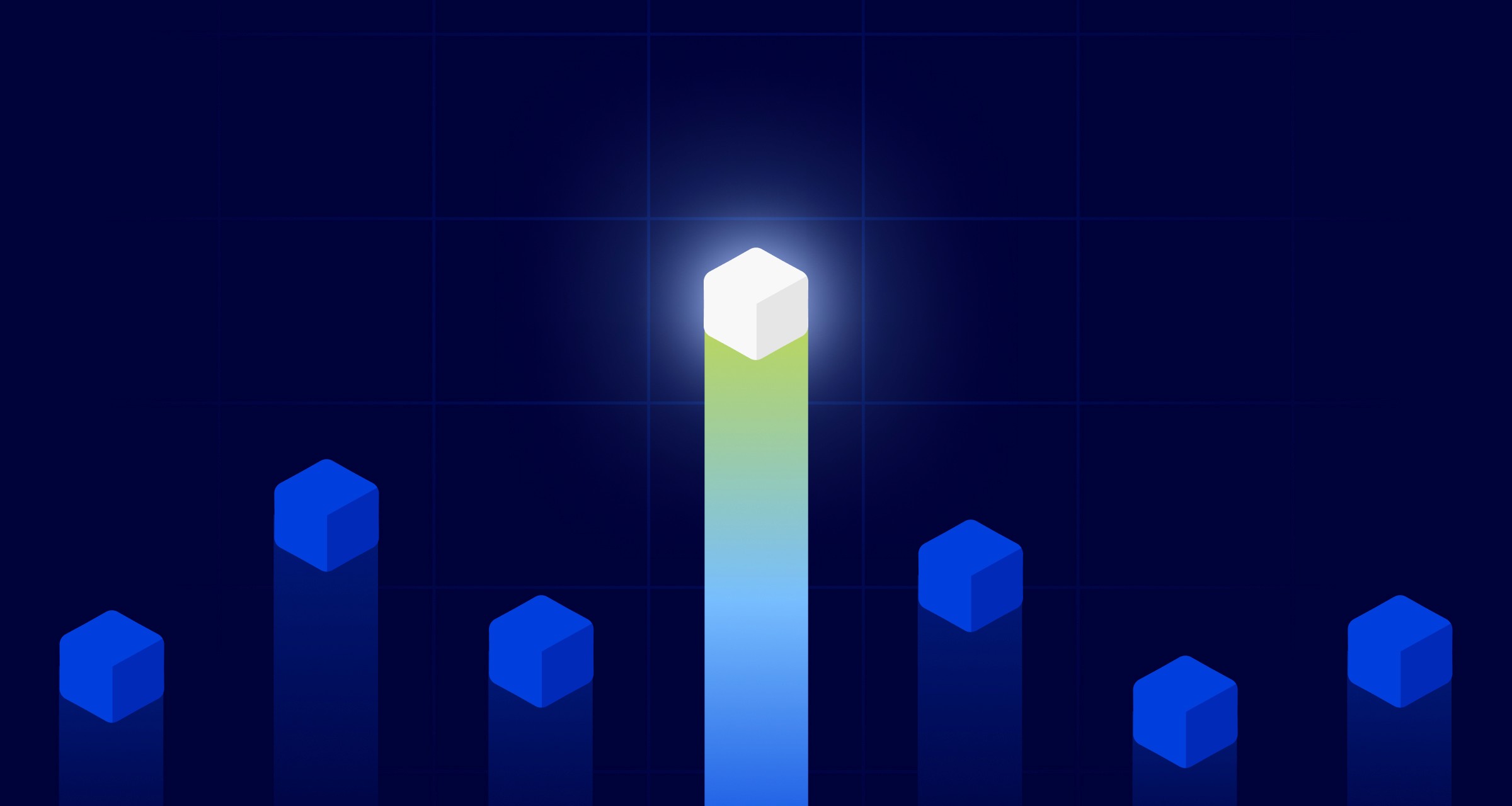
'Align to Innovate' benchmark: state-of-the-art enzyme engineering with fully-automated GenAI
'Align to Innovate' benchmark: state-of-the-art enzyme engineering with fully-automated GenAI
'Align to Innovate' benchmark: state-of-the-art enzyme engineering with fully-automated GenAI
Oct 3, 2024
Oct 3, 2024
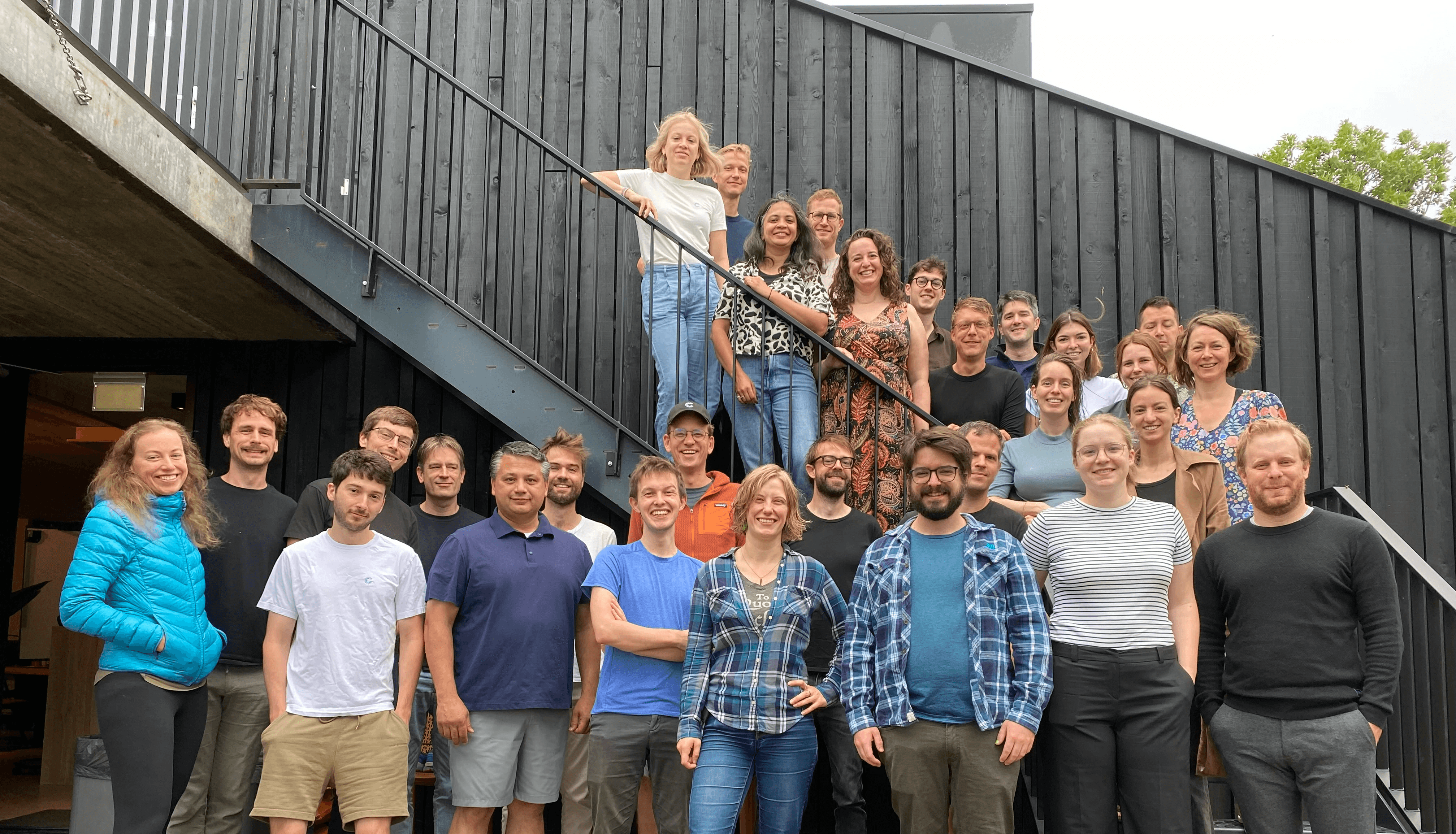
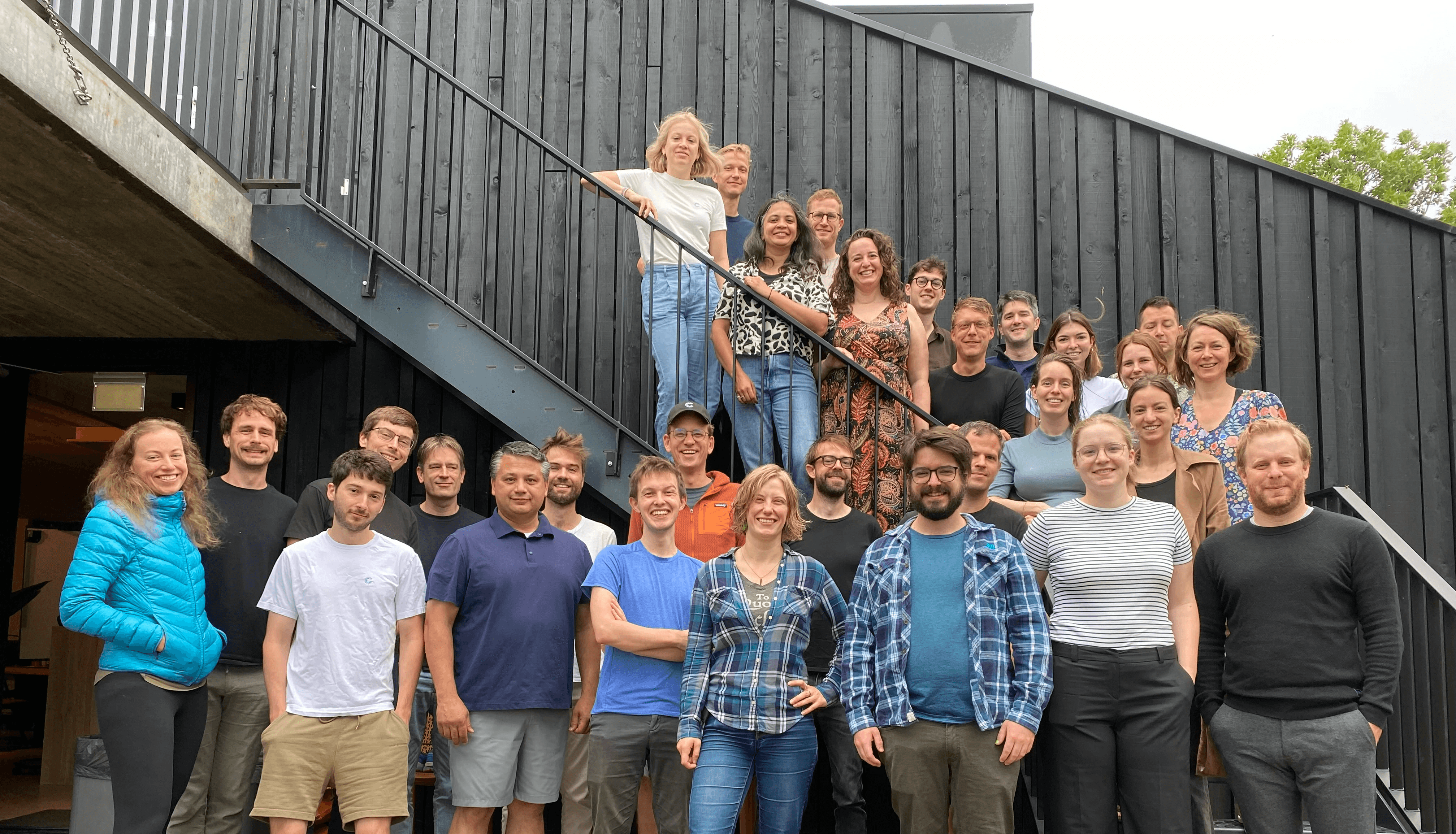
Cultural values at Cradle
Cultural values at Cradle
Cultural values at Cradle
Oct 2, 2024
Oct 2, 2024

Stay in the loop
Stay in the loop
Stay in the loop
Get new posts and other Cradle updates directly to your inbox. No spam :)